The powder keg of the modern data stack
The thing about a single pane of glass is that there can only be one pane of glass. Plus, survey results!
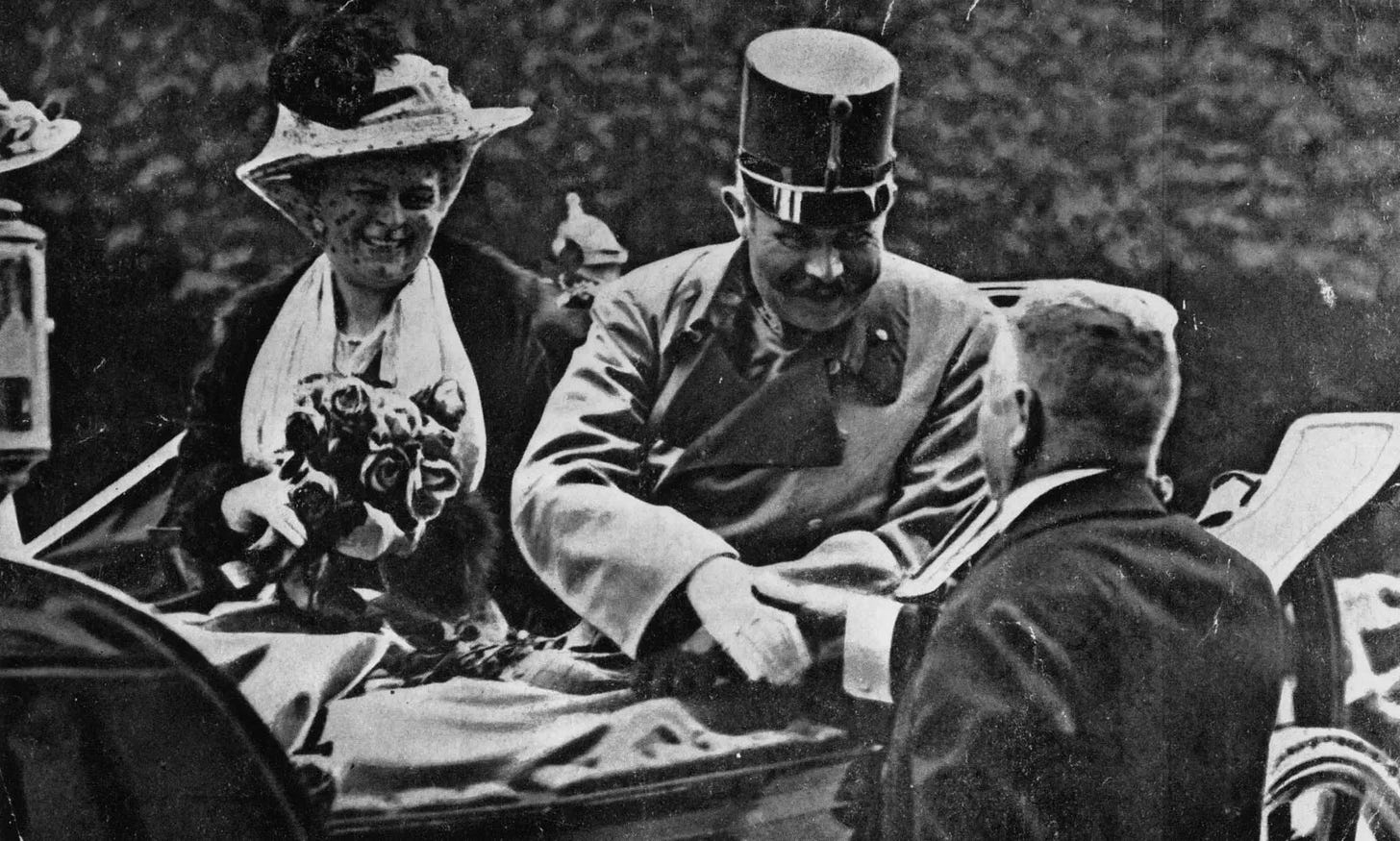
It's 2032, and the halcyon days of the modern data stack are over. The funding environment has cooled; TechCrunch has moved on to the next new thing;1 community conferences in Austin have been replaced by trade shows in Dallas and the “cool” newsletters have been replaced by CDO Weekly, sponsored by Microsoft Synapse. The data startup ecosystem no longer looks vaguely like a Ponzi scheme, in which data teams build internal tools; then start companies based on those tools; then hire their own data teams; then those teams build more internal tools; then they create more data companies; and then we all sell our products to one another. Instead, data teams just buy a handful of boring defaults, and move on. Those of us who are still working in the industry tell war stories about this era, and the youths laugh at us, behind our backs.
In this calmer world, we've all stopped arguing about semantic layers, data contracts, and how we make sense of what all of our data means. In some improbable twist of fate, we landed on the good timeline—data is no longer scattered across everything, everywhere, all at once.2 In our lucky fragment of the multiverse, data is organized and manageable. Entities are cleanly defined. When we look at dashboards, we debate what we’re going to do about them, not if we can trust them. When we send marketing emails, we wonder which segments we should send them to, not if we sent them to the right people. Our tools all work in seamless harmony.
Suppose that you’re an executive in this blessed cosmos. A new director joins your team, and, being the analytically-savvy leader that we’ll all need to be, they ask where they should go to start getting their arms around your company’s data. They want to get a bird’s eye view of what data is available, the sources it’s drawn from, the key assets that use it, and the health of the whole system.
What specific website do you tell them to go to?
Back here in 2022, there’s no one place to go for this information. Our data tools are now infamously fragmented, and our answer to the director’s question would be a list of a half-dozen vendors and a likely out-of-date wiki that’s sporadically maintained.
We can all agree that this isn’t exactly ideal. Life would be much easier if we had a single source of truth—or a “single pane of glass,” if you prefer corporate technobabble—for what’s happening across the data stack.
But to get there, we also have to agree on who should provide that pane of glass—and right now, there’s nothing close to a consensus on that question.
Eyes on the same prize
For the last few years, most data startups followed Peter Thiel’s advice: Avoid competition. Companies have consistently tried to carve a unique niche for themselves, looking for some bit of white space in the data landscape that lets them be Switzerland—nobody’s competitor, and everyone’s partner—to as many other players as possible.3
This positioning, however, can’t last forever. Eventually, as startups grow, companies that see one another as polite partners will start to jockey for the same space.
Collisions like this are happening in a few places, though each one is at a different stage. Major warehouse vendors like Snowflake, Databricks, and BigQuery have already declared war. ETL vendors (forward and reverse) are squaring up, and have had a few skirmishes. BI and analytics companies, which are still running around like a thousand different bickering tribes, haven’t drawn enough categorical borders to have an organized fight, though one is surely coming.
The biggest looming battle, however, will be over a different territory: The brain—or operating system—of the data stack.
Nothing like this exists yet. Nothing can tell us about the various activities that are bouncing around in our data tools, much less coordinate and manage that activity. Nobody owns the logic that orchestrates data services, or governs how different products talk to one another. There is no good answer to our director’s question from 2032: “What is the management console for our data stack?”
But it would be nice if there was one, and I suspect a lot of companies have already started eyeing this idea. Data catalogs, data discovery platforms, metrics layers, observability tools, transformation and orchestration tools4—all of these categories offer a sliver of centralization, from a single place to find data, to understand its lineage, to monitor its accuracy, or to define the business logic that’s applied to it. It’s easy to imagine a three-step master plan from each of these companies, starting with where they are today, and ending with being the control tower for the modern data stack.
More importantly, for a lot of companies, this roadmap may not be a loose vision, but a fiduciary promise. Categories like data cataloging are probably too thin to support the hundreds of millions of dollars in revenue that companies need to find to justify the investments they’ve taken—to date, the fourteen startups listed above, to say nothing of the those that aren’t included, have collected a total of $1.2 billion in funding. That will lead them to chase new markets, and the obvious choice is the very valuable opening at the center of the data stack. If that’s the case, a half dozen categories and scores of companies are careening toward a massive collision.
It’s always a people problem
None of this is exactly news. Collisions like these are inevitable in frenetic markets, and this case is no different.
Still, I think it’s remembering this dynamic when we criticize the state of the modern data stack, and the apparent chaos within it. More than anything, the problem isn’t our technology or how teams use it; it’s the market. The master of ceremonies is a valuable role to be, and lots of companies have probably recognized that the data stack doesn’t have one yet. That discourages us from rallying around any of the fourteen potential standards linked above, and instead pushes each of us to create a fifteenth.5 Generally speaking, if we can’t get something right in that many tries, the issue probably isn’t some tweak that we’ll fix on the next one. The issue is systemic—and each new effort to make it better actually makes the fragmentation worse.
So where do we go from here? For better or for worse, the market giveth this problem, and only the market can taketh it away.6 But we can help it do its work, I think, by more openly acknowledging the reality of the situation: There are a lot of companies that are implicitly competing to unify the disparate elements of the modern data stack.
The companies caught in this traffic jam should evaluate their products and roadmaps against their chances at winning that market, not the small box they currently compete in.7 And if their odds are low—which, for a lot of companies, they probably are—I’d argue that they’d be better off figuring out how to thrive if someone else owns the center of the data stack. Because by 2032, someone will own it. But there can only be one unifier—there can only be one single source of truth, one single pane of glass—and not everyone can be king.
Reader mailbag! – Are companies profitable?
Four months ago, I wrote a post that included a survey asking people how frequently their employers actually turned a profit. A day late (and in this survey’s case, many dollars short), here are the results:
Thirty people responded to the survey. In total, those people have worked for 361 years. In 166 of those years, or 46 percent, people’s employers were profitable. There wasn’t much difference between people who identified as a tech person, a startup people, neither, or both. In every group, companies made a profit 45 to 50 percent of the time.
However, people with more experience had spent a lot more time at unprofitable companies. People with less than ten years of experience spent 73 percent of their working years working for a profitable company. For people with ten to nineteen years of experience, that figure fell to 50 percent; for people who’ve worked twenty or more years, it fell even further, to 26 percent. I have no idea what to make of this, other than to assume that there’s a lot of bias in the sample—if you’ve been working for thirty years and made money in most of them, you probably have better things to do with your time than respond to surveys on some some weird blog.
Finally, to those of you who had something else you wanted to yell about:
—
The rent is too damn high, but if I move where am I supposed to get good pho at any hour of the day.
This survey was in March, so at least rents haven’t gone up sinc—oh my.
—
I assure you the impulse to elevate data and analytics teams to be "strategic" and to own decisions and sit in board meetings, etc is negatively correlated with profitability.
Look, the rent is too damn high, and some of us need to make sure the music doesn’t stop. Let us tell ourselves that all of this angst is just imposter syndrome; we’re worth it; I’m doing great; we’re all doing great.
—
Hillary Clinton’s emails.
Yeah, but what about Hunter Biden’s laptop?
—
Benn with two n's is a great brand.
Shoutout to Heather and Jim for that one—a great teacher, a great lawyer, great parents, and now, great brand marketers.
Like, I dunno, B2B SaaS products for companies that exist solely in the metaverse. Don’t tell me that between now and 2032, someone won’t pitch their startup as Shopify for Roblox entrepreneurs.
What paper cuts do I have to give myself to jump to this timeline?
In fairness, this isn’t just about how companies position themselves. The market is unsettled, and a lot of buyers purchase tools that appear, on the surface, to be directly competitive.
I’m a personal investor in Bigeye and Elementl.
I’d argue that this dynamic also explains why we still don’t have any good analytical templates. Most template libraries are created by vendors—be it Mode, Looker, Tableau, Sisense, Deepnote, Hex, Streamlit, or someone else. Sure, there are well-meaning community intentions behind these efforts, but they’re primarily meant to drive a business outcome. This means that companies are motivated to promote their templates over templates as a general asset. It’s tough for a standard package of templates to emerge when everyone who’s creating them would, if push came to shove, probably knife someone else’s library if it gave theirs a better chance to succeed.
Will it work through its invisible hand, a papal bull from on high, or Stripe’s hitmen? Time will tell.
When companies in these various categories raised money, I suspect most of them didn’t show vendors from other categories on their mandatory two-by-two competition matrix slide. But if they drew the same grid for who they’d be competing with at the end of their path to $100 million in revenue, there’d be a lot more overlap.
I feel like the ultimate elephant in the room is having a unified understanding of data across all stakeholders of it (so that you can better communicate, operate, etc.). This includes the business-facing, data scientists, data engineers, etc. I agree that an unified pane will provide a much needed management console for these people. I further believe this management console is one potential solution for this bigger knowledge issue. However many of the data products tackle this issue by selling their products to the top (i.e. head of data) who then enforce the product on their subordinates. I'm curious on your thoughts of the reverse. Create products that individuals like data engineers use regularly in their workflows. Then aggregating all of this into one cohesive console - creating a sort of hive mind understanding of data at the end. Not sure if there are any examples of this out there.
I see external factors also influencing the 2032 reality or the possibility of a single view of data For eg. 1) Source data (Telemetry) to a large extent is not yet standardized, do we see more source problems in 2032 meta world or more standardization. 2) Would enterprises substitute a large part of their microservices with SaaS tooling (eg. A world where companies always use stripe and its competitors for payments, with similar export schemas a.k.a data cloud), or would they continue building their own in-house services (may be they wish to avoid overhead of managing too many vendors, legal, privacy and procurement issues)