The case for being biased
Why telling the truth might be the second-most valuable thing data teams can do.
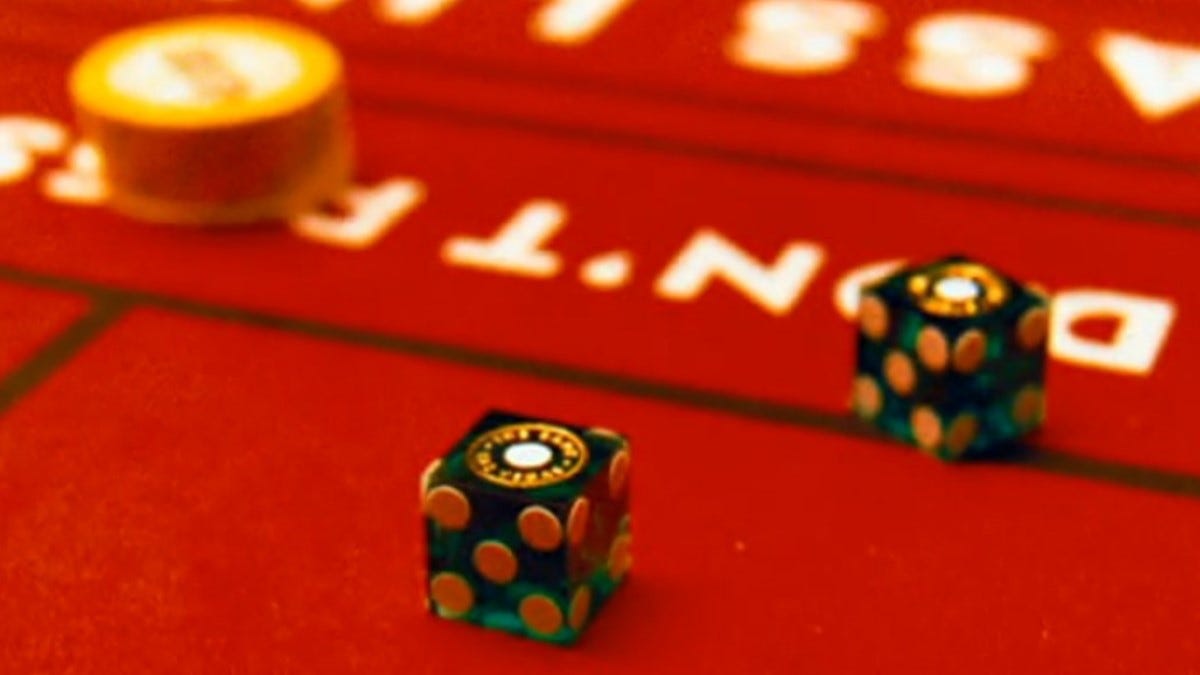
While we're on the subject of the all-time greats, I’ve got two candidates for the best interview of all time.
The first contender is an engineer that we interviewed at Mode in 2015. He was at our office for the onsite, and we were prodding our way around his resume, asking canned questions about his prior jobs. In the middle of deadpanning through an otherwise unremarkable answer, he trailed off, his eyes drifted into the middle distance, and, as if sabotaged by some microscopic rebel, his brain sputtered, stalled, and powered down. We all froze in the silence. After a few seconds, he lurched back to life, and said, as flatly as before, "Oh, sorry. I just got really excited about the internet of things."
We tried to resume the conversation, but none of our hearts were still in it—his stolen by the possibilities of wifi-enabled thermostats, and ours unsettled by the visitation we'd just witnessed. Later, when the interview panel got together to make a decision, we learned that he'd apologized in another conversation for “doing too many drugs prior to the interview.” Networked hardware is a hell of drug, I guess.
The second candidate for the greatest interview ever—and my personal favorite—had applied to be a data analyst at Microsoft. We were always hiring for this role, so the phone screens were standardized and formulaic. We typically opened the call with a few simple math questions to judge how comfortable people were with thinking through and explaining quantitative concepts. This interview started just as hundreds had before: "If you rolled a standard six-sided die and got paid based on what you rolled—for example, roll a three, get three dollars—how many dollars would you expect to make from one role?"
"Six," he said.
"Six? How did you get six?"
"I'm the kind of guy who rolls sixes."
Never in my life will I say something so incredible. The stunning certainty; the breathtaking audacity; the complete disregard for the assignment. He was Banksy, humiliating us and everything that we thought was valuable; he was James Harden, leaving us twisted and broken on the floor; he was our nemesis, a criminal mastermind flawlessly orchestrating our every helpless move. In one cavalier wave, he dismissed lifetimes of careful study about uncertainty as a cowardly distraction, a brash and bullying alpha to our pocket-protected beta.
He was also, of course, completely wrong.1 No amount of confidence will make him more likely to roll a six. No amount of genius will unwind the impossibly complicated physics of a tumbling die. God may not play dice, but for us mere mortals, probabilities are the best we can do. As analysts, and as candidates interviewing to be analysts, that’s our job—measure the odds, do the math, devise a strategy, and hope the dice land the right way.
Or is it? In my recent descent into data team existentialism, I’ve started to wonder if he was actually onto something, and it was me who was misunderstanding our assignment.
What’s the truth for?
If all the various flavors of data philosophies were different sects in a religion, the search for understanding and truth—for the real probabilities in our corporate craps game—would be our shared savior. No matter the denomination of the data team—reporting specialists, internal consultants, builders of data products, a help desk, insight seekers, coin-operated mercenaries—we all agree that our jobs are built on objectivity and honest observation. Our very name, data science, suggests that we’re on some noble quest for worldly knowledge and apolitical enlightenment. Even when we criticize that term, our objections are to our unscientific methods. On our goals, we’re unequivocal: We’re here to figure out what’s true and tell people about it, unbowed by bludgeonings of organizational politics and executive pressure.
But, crass as it may sound, truth is just a means to an end—to make a company successful. We aren’t paid to be curious researchers who are trying to understand a confusing world; we are, just like every other employee, corporate instruments for making money. Our desire to find the truth is a retrofitted projection, built on the assumption that data begats discoveries, that discoveries begat knowledge and understanding, and that knowledge and understanding begats business success.
Put differently, the fundamental argument underneath most data teams is this: Given the skills we have, we can contribute to our employer’s success by figuring out what is true. Though that seems obviously right, it answers the wrong question. The question shouldn’t be if this is a useful role for a data team; the question should be, is it the most useful role for a data team?
Trust the process
In 2013, the perennially mediocre Philadelphia 76ers hired Sam Hinkie as their new general manager. Almost immediately, he put in place a now infamous plan: Rather than staying stuck in the middle of standings, he’d intentionally tanked the team by trading away its top players for draft picks. They’d then finish at the bottom of the league and get more top picks, which they could use to draft a handful of future superstars. Eventually, they’d collect enough young talent to not only be a contender, but to be great.
In its early days, the plan was predictably controversial. For Hinkie, this wasn’t just a PR problem; it was an existential one. Doubt from players and team executives could undermine the entire effort. Players and coaches, for example, might get frustrated during the team’s dark years and demand trades. Hinkie’s job, then, wasn’t just to devise the strategy and to execute on it; he also had to sell it. And sell it he did: “Trust the process”—the shorthand for the plan—became a catchphrase, a brand, a lifestyle.2
During this critical moment, after players had been traded away and The Process was in motion but before people were convinced it’d work, what should the 76ers' data team do?3
Should they be reporting the facts to Hinkie and the rest of the organization, and be bold truth tellers about what is and isn’t working? Or should they be selling the strategy, and doing everything they can to make people believe in it? Should data be for explaining nuance, or should it be propaganda, used selectively to keep people committed to the now irreversible plan?
This dilemma highlights the key difference between business decisions and scientific ones, and between what analysts and actual scientists do. For the chemist in a pharmaceutical lab or the physicist in a wind tunnel, natural truths matter. Will the chemical compound eradicate the disease, or poison the patient? Will the plane fly, or not? The answers to these questions, like the outcome of a roll of a die, aren’t affected by the resolve of the doctor, or the confidence of the pilot. Physics and biology will cure the sick, not courage. The laws of aerodynamics will keep a plane in the air, not faith.
The success of business decisions and strategies, by contrast, depend heavily on what happens after a decision is made. To stick the landing, you’ve got to commit to the jump. Execution and organizational alignment often matter as much as the decision itself.
Data can be a powerful weapon in this operational struggle. Data is deeply persuasive, especially among today’s quantitatively conceited corporate class.4 When woven through tight narratives, it can paint clear pictures of where a company wants to go, how it will get there, and why the chosen path is the right one. It can keep people from walking at different cadences, or from questioning if they’re going the right direction at all. Data can even tap into baser instincts. Few things will make us as manically obsessive about something as much as regularly measuring it.
In this light, it seems at least possible that the 76ers’ data team—and by extension, every data team—would be more valuable as periodic propagandists than they would be as truth seekers. As the cliche goes, the man who thinks he can and the man who thinks he can't are both right. The same principle surely apply to organizations as well. While waffling and second guessing can undermine even the best strategies, flawed plans can be overcome by a commitment to make them work.5
Admittedly, this doesn’t feel right. But I think that reaction is mostly emotional, and my objections to data teams leaning into biases rather than away from them don’t really hold up.
It’s immoral
Using data in this way sounds perverted if not outright profane, improper if not outright immoral. But is it immoral for a CEO to give a rousing speech to rally a company? Is it immoral for a marketing team to cherry pick numbers to sell a product? Is it immoral for startup founders to aggressively promote their company in a pitch deck to a venture capitalist? At best, we’d say no, this is what they should be doing; at worst, we’d say the answer is complicated. In all these cases, we’d recognize that there are times to put our thumbs on the analytical scales, and to be selective about the truths we tell.6
Data teams don’t operate on a higher moral plane than these other functions. We’re here to promote the same outcomes as everyone else. If we can support those goals—by presenting, say, a compelling but biased analysis—why should we be exempt from doing so?
People would see through it
If we stop being objective all the time, data could lose its persuasive power. Practically speaking, though, it probably wouldn’t. People aren't all that critical of data or analysis, especially when they aren’t motivated to doubt it. What’s more, it may not matter if people knew some bit of analysis was more partisan than balanced. People are affected by facts and narratives, even when they’re told they’re completely made up and they’ve been explicitly misled.
Professional wrestling is the ultimate proof of our willingness to surrender to things we know aren’t real. Wrestling is cartoonishly fake. It panders to our most ridiculous indulgences. But it gives its fans a chance to elope to an imaginary world that seems more fun than the one they’re in, and they happily go along for the ride.
In what’s probably the only parallel between business intelligence and the WWE, data can provide a similar escape. A few compelling numbers give us permission to see the world as we want to see it. Even if we know they’re not entirely honest, they tell us that it’s ok to believe.
Somebody has to be the referee
Certainly, sometimes companies need nonpartisan researchers and unbiased scorekeepers—I’m not arguing that we should give up this duty entirely. But I think our insistence to always play that role is mostly self-serving. It’s cooler to be a bold contrarian than a patsy for the party line. It’s easier to lightly question every decision, so that you can stay silent if it goes well, and be able to say “I told you so” if it goes wrong. And there’s power and comfort (and a sense of smug superiority7) in working above the political fray. The more we can convince ourselves and other people that we’re here to be fact checkers and not pundits, the more unimpeachable our influence—but the less useful our impact.
I believe that we will win
In her most recent post for the Analytics Engineering Roundup, Anna Filippova asked a question: “What business scenarios require information delivered within minutes?”
I found myself wincing at her answer. She said, for dbt Labs, they need minute-by-minute data on product launches and the deals that are closing at the end of the quarter. Why, I thought, would they possibly need this? What decisions do they need to make that would justify the work it must take to cut a sales dashboard’s latency down from three hours to thirty seconds?
But Anna, like the analyst we interviewed ten years ago, is working on an entirely different plane than I am. The point of the dashboard isn’t to make decisions. It’s to keep the mission at the front of everyone’s mind. It’s to make sure everyone sees the impact of the work they’re doing. It’s to rally a company around a sales team pushing through their final sprint. It’s to use data, not to tell detached truths, but to connect with people emotionally, to make them trust the process, and to help them believe, despite all the odds stacked against startups, that theirs can go out and hit a hard six.
Wrong, I should say, in the way that Van Gogh’s stars are wrong, or Turner’s trains are wrong.
The plan mostly worked. They were indeed terrible for a few years, and finished with one of the worst records in the NBA for four straight seasons. And then got way better. In 2021, they had the best record in the Eastern Conference.
Hinkie was also famously analytical, and the 76ers actually had a data team at the time. So I I ask this question hypothetically, but also, if anyone knows what that team did, I’d love to hear.
My general theory is that most people want to be able to say that they make decisions based on data and sound reasoning. Many don’t—they start with their preferred outcome and work backwards from there—nor do they care that they don’t. But appearances are important, which is why quantitative arguments are so persuasive. We feel safer when we’re armed with one.
Sounds like a cult, you may say. And, yeah, fair, but cults…work? (Counterpoint.) I’m not going say that I’m pro-cult, but cults have been able to accomplish—if you use “accomplish” in a very loose sense—a lot of unbelievable things (literally; it is hard to believe what cults make people do). I’d guess that most successful startups—and maybe bigger companies, though that seems less obvious—are successful in part because employees develop a cult-like devotion to their product, mission, founders, or culture.
Importantly, this is different from outright lying. You can be persuasive without being dishonest.
As the youngest child, I live for this. Nothing gives us more self-righteous satisfaction than sniping from the sidelines, all while pretending to be a neutral bystander.
Benn, how did you break into my brain and steal the article I'm currently writing?
I think this is such an important insight about data that I've personally struggled with. The action our data inspires is as much or sometimes more important than the truths and insights it reveals. We can sigh and say a dashboard objectively worthless all we want, but if it makes people happy and focused, it's having a material impact.
Great post, Benn, definitely hit on some key points and issues with value-add. There may be some areas where we might slightly disagree in terms of terminology and characterization. As someone who has worked in both the quantitative and qualitative sides of analysis, I've come to appreciate the value of each tool set, and I think there lies some of those issues you describe.
I think the main issue you are highlighting is that some data team are too strict in their adherence to what the data says (both in input and output) and perceived objectivity, while ignoring or discounting context, intuition, opinion/judgment on the principle that they are some sort of *bias*. We forget that data isn't everything, and there is value in qualitative analysis and analyst judgment. Things like argumentation, pragmatism, intuition, and context. At times, some data teams may lean too heavy on their data tools and discount the value of qualitative analysis. I'd argue it's more "lean into the squishy stuff" rather than "lean into bias".
I don't think we lose our role as truth seekers, but rather, we should not lose sight that we are a part of the rest of the company, aligned with the strategy and policy put forth by leadership and the data and analysis that is produced should support that strategy or policy, but always honest about the performance of that strategy or policy.
Not everything can be explained via clean data or the results of a model, but there is value in analytical judgment. Data teams develop a deep intuitive understanding of the underlying subject matter that comes from wading knee deep in the data day in and day out, and should lean into that more. There is deep and useful knowledge in the minds of the data team that can be synthesized qualitatively and with analytical judgment and in conjunction with leadership and company strategists.
In terms of Hinkie's strategy, I don't believe the data team should be "selling" it, per se, but rather providing analytical support to help decision-makers understand its progress. This requires being selective and using analytical judgment to determine what information is most relevant to share. We need to be analytical entities working in conjunction with decision-makers to provide them with the truth, or as close to it as possible, to ensure success.
While we may discount initial reports showing a decline in performance, we should still provide them with the analytical caveat that this is expected in the initial stages of the strategy and it is important to recognize that it may take time before we start seeing actual signals in the data.