Do we still need the world wide web?
Why fast—and not free—could reinvent the entire internet. Or at least, I dunno, data catalogs.
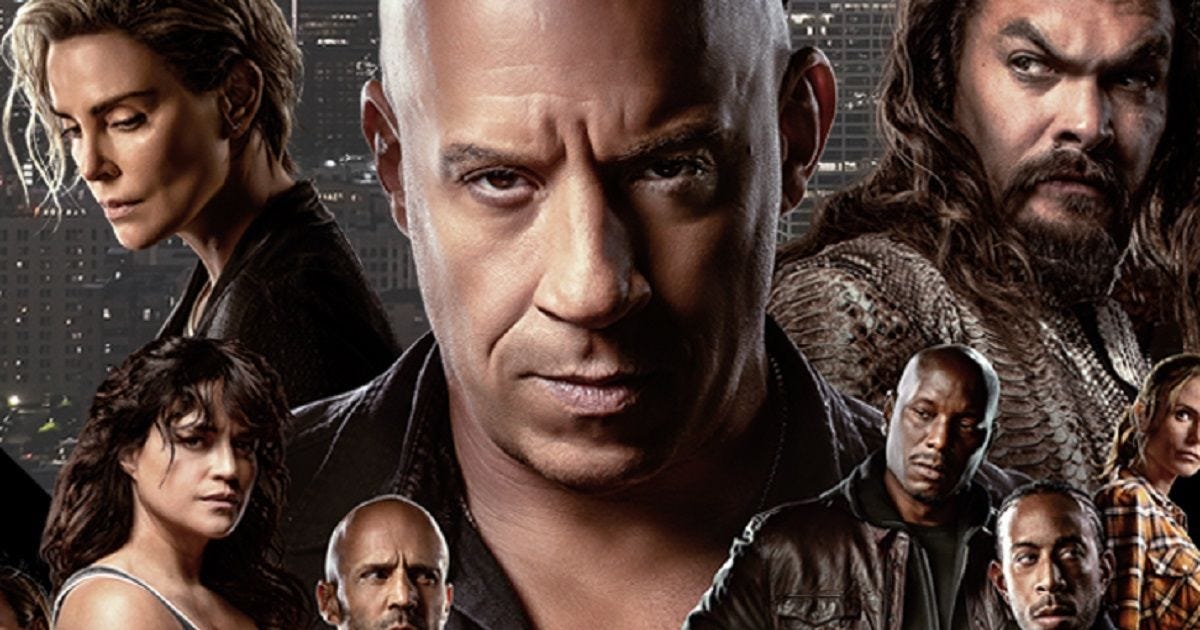
We haven’t yet agreed on whether or not AI is going to destroy humanity, but there seems to be a growing consensus that it will destroy the internet. As the cost of content creation falls to zero, the story goes, generated content will overrun the web. Soon, every site will be a soulless cesspool of clickbait, inbred on top of a spiraling gene pool of derivative junk mail and content marketing.1
This dystopia seems plausible enough—it is, after all, already happening. YouTube factories have existed for years, retching out thousands upon thousands of horrific videos, hoping that one—maybe the one that combines Peppa Pig, Easter eggs, and, I don’t know, being buried alive?—catches an algorithmic updraft.
Historically, we’ve been partially protected from these sorts of content chop shops for both technical and social reasons. Algorithmically-created articles, images, and videos were just hard enough to produce and of just low enough quality that their creators had to fully commit to the bit. Using this stuff was roughly synonymous with content farming; content farms are seen as a kind of sleazy grift; legitimate enterprises aren’t cons. So, in our playing of the Great Online Game, we had to choose: Be a respectable creator and hand-crafted our content, or run an internet tourist trap and sell junk.
OpenAI, Midjourney, and the tsunami of interest in generative AI has changed all that. The technologies make it easy and inexpensive for anyone to create content; the hype makes it not only socially acceptable to do so, but foolish and short-sighted not to do so. Every other word in company keynotes is generative AI; every third question on earnings calls is about it; every fourth tweet is an urgent thread warning us that, unless we subscribe to a weekly newsletter on the latest prompt engineering tips, we’ll soon be fired and replaced by an intern and a chatbot.
And so, the thin levies between us and an unending sea of spam are starting to break. BuzzFeed is experimenting with AI-written articles. DJs, from David Guetta to anonymous people on TikTok, are charting with AI-generated songs. As these things get cheaper and easier to create, the rest of what we consume on the internet must not be far behind.
Starting today, everyone loves documentation
Anyway, earlier this week, Atlan, a data catalog company,2 announced Atlan AI:
Starting today, everyone loves documentation.
The importance of documentation cannot be emphasized enough, especially with a lot of teams operating in hybrid or remote environments. But documentation of data, especially when done manually can be a tedious experience.
Atlan AI is set to automate the documentation process. It can document hundreds of assets in just minutes, making it especially helpful for those who have just started their data governance journey and have a backlog of data assets with missing documentation.
As the launch site says, today, every dataset or table in your warehouse has to be manually documented; soon, Atlan AI will do it for you, cranking out hundreds READMEs with a single click.3
One theory you could have about this is that it’s very bad. It could be further proof of the inevitable cheapening of digital content, and the beginning of us polluting our internal documents with errors and inaccuracies. Even if generated content is meant to be edited and reviewed by a person, it’s unlikely to stay that way. “Drafts” will leak through, or people will mechanically approve articles while multitasking through Ted Lasso.
A second theory you could have is that it’s very good. A notorious problem with data catalogs (and probably any other sort of similar internal resource) is that someone has to author and maintain the catalog. It’s a thankless task that few data teams have any desire to do, and is precisely the sort of job that interns are asked to grind through—and therefore, is also precisely the sort of task that’s well-suited for an unfeeling, unsleeping, unpaid AI.4
But a third theory you could have is that it doesn’t make any sense. If a computer can manufacture documentation on the fly, what’s the point of writing it down at all? Why dutifully record a bunch of notes about something if I can conjure a new—and presumably, more up-to-date—version of it whenever I need it?5
One very reasonable answer to that question is we have to write it down because we’ve long assumed that documentation would be written down. We don’t look things up by asking questions, not exactly; we look things up with search, and that only works if we have something to search for. And so, at least in the short term, Atlan automatically creating documentation very much makes sense.
But that default to search—long-standing though it may be—isn’t fixed. As we become more accustomed to a world where things can be made in an instant, the immediate, on-demand answers from Atlan’s chatbot assistant may be all the documentation we need.6
The fast and the free
The point here isn’t really about Atlan, or even data documentation. It’s about the distinction between free content creation and fast content creation. When we talk about the effects of generative AI, we tend to project forward from the idea that it’ll be cheap to make stuff. For better, we’ll make more and a wider range of things; for worse, we’ll mass-produce creative content just as we mass-produce physical goods. To imagine our future, we imagine a world full of factories for manufacturing cheap creativity.
If that’s where this is all headed, it’ll undoubtedly change a lot of how the world works. However, I’d guess that the more interesting effects will come not from the creation cost of content falling to zero, but from the creation time falling to zero. Consider a handful of examples:7
If all shipping were free, we’d buy more stuff online. If all shipping were free and same-day, we’d shop differently, by buying lots of things to try out and return. We’d no longer shop for clothes or furniture; we’d test and lease everything.
If Google was free but took as long to return a result as looking it up in a book, we ask it questions a few times a day. But because Google is also instantaneous, we look up everything, from restaurant recommendations in our home town, to how we get to them (despite having driven there several times), to how much to tip on a $78 check.
If running data tests or a code linter were free, we’d run more of them, but develop in the more or less the same way. If they were both free and immediate, we’d redesign our data pipelines or our development workflows around them.
If training new employees were free, we might hire a few more people, since the net cost of every employee would be a bit lower. But if people could be fully trained the day they started a new job, we’d completely change how we hire, taking more chances on risky candidates and potentially replacing interviews with day-long on-the-job trials.
If air travel were free, we’d all probably take more trips, but many of us would still live in the same places. If air travel was replaced with free teleportation between airports, the entire notion of physical distance would get upended. We’d scatter ourselves across the countryside and around the world. We’d live in Paris, work in New York, and spend our weekends in Tokyo.8 The very idea of citizenship would start to erode.
In other words, price changes mostly affect how much of something we consume. Speed changes how we consume it—and the effects of that can be much more profound. The same principle seems to apply to content generated by AI:
Per the Atlan example, if documentation were free to create but still took hours and days to write, we’d probably just write a lot more documentation. If it were free to create and could be created immediately, we’d get rid of our data dictionaries and replace them with a service for answering questions on demand.
If answering an analytical question was free but still moved at the same speed as a request working its way through a data team ticket queue, we might ask a few more questions. But if we got an answer back as soon as we asked it, we’d investigate everything. Analytical bots would be in meetings what Google is at a bar: The immediate arbiter of any dispute. Moreover, just as it was Google—and not a free library card—that made us all amateur researchers and historians, it’s the instant feedback loop between question and answer that would finally make us all the “citizen analysts” we’ve been hyping for years.
If ad content is free to create, we run more tests through the same ad infrastructure that we use today. If ad content can be created the moment the ad gets served, we begin tailoring ads to individual buyers and moments, and inserting them into content natively.9
And more generally, if content on the internet is free to create, then people will build assembly lines to crank out traps for Google searches. But if content is free to create and can be created immediately, people stop searching. They either go to the destinations that they know, or directly ask for the answer they need.
This is why I’m skeptical that generative AI will turn the internet into content mills like eHow and Answers.com. These sites can only dominate if we continue consuming content in the same way—search, click, search, click. Imagine, though, what the future of looking for a recipe might look like. Today, we search for “sprinkle sugar cookie,” get a million results, choose the first result from a reputable-sounding domain, scroll past the childhood story about maple syrup sugarhouses in Vermont, and decide if this is the recipe we want to make. As ChatGPT, Bard, and Bing’s chatbot get better, we’ll either go straight to our favorite site, or we’ll ask the bot for a recipe. Don’t have an ingredient? Instead of searching again, we’ll ask it to make a substitution. Mass-produced recipe sites don’t proliferate; they go away. And the infamous stories that come before the recipes? They’re there for Google, and probably go away too.
Though this is a small example, I think it’s illustrative of how the longer-term effects of generative AI may not be to produce more internet, but a different internet. So much of what exists today is built on the almost-now-invisible assumption that content takes time to produce, and search and social media the primary arteries of an overwhelming majority of traffic around the web. Unless this AI circus is a giant bubble, my guess is that neither of those assumptions will be true for much longer. And I’d bet the companies and products that are built for that world—that extrapolate their visions of the future from the idea that content will be immediate as much as content will being free—are the ones that will ultimately be the movement’s big winners.
One hilarious example: This launch video of Copilot in Viva Engage. (Lest you think I routinely watch marketing videos for obscure Microsoft products with names as compelling as Viva Engage, I came across this video because Viva Engage is what Yammer, a product I used to work on, turned into.) In the video, our corporate protagonist is, first, recommended to “join the conversation” about a food drive at her company; second, given six paragraphs of machine-generated text for her post; and third, handed a few links and pictures to attach to it. At the end, she adds her only contribution: A single sentence that says “this cause means so much to me personally.” It sure seems like it does, Carole.
Data catalogs are basically encyclopedias for data assets, like tables and dashboards. Want to know what the “sector” column means on the “customers” table, or which dashboards use data that’s derived from Salesforce? A data catalog can (theoretically) tell you.
The upcoming release includes a hefty list of other features, like natural language search, a data discovery virtual assistant, a SQL interpreter that explains SQL logic in plain English, and a chatbot that can turn a question into a query.
I do wonder if being polite to ChatGPT generates better responses. It doesn’t seem crazy to think it would? On Google, I’d guess that pleasantries like “please” and “thank you” could make a search query fuzzy and imprecise, and lead to worse results. But on ChatGPT, this could tilt the model towards responses like those in which people had been polite in the training data. For some questions, like asking how to do something, that nudge could be helpful. (That said, for others, like asking for a good joke, I could see it actually producing worse responses.)
An analogy for the geriatric millennials: When school lessons were entirely analog, every statistics textbook had dozens of conversion tables printed in the appendix. Whenever you wanted to convert a z-score to a p-value, you had to look this up in the back of the book. Get a z-score of 1.9433; round it to 1.94; flip to table C1; go to the row for 1.9; to the column for 0.04; get a p-value of 0.9738. (Then, subtract this from one to get 0.0262; double it to get 0.0524; throw out some “outliers” and “unreliable observations” until you can nudge it under 0.05.)
It was an imperfect system. Because publishers could only dedicate so much space to these tables, they had to be truncated. You often could only look up values at important thresholds (e.g., for p-values of 0.1, 0.05, and so on), and had to estimate your result by extrapolating between them.
Computers could’ve solved this problem in two ways: By creating a giant scrollable table with far more lookup values, or by letting people type in their input values and getting rid of the tables entirely. The second choice is obviously better. And that’s true even though these values are mathematically fixed. If the corresponding p-value for a z-score of 1.94 was constantly changing—sometimes it was 0.9738, sometimes 0.975, sometimes 0.9, sometimes null, and sometimes down for maintenance while we figure out why it was null—the second option only gets better.
There’s also another reason I’m bearish on the long-term value of traditional data catalogs. Roughly speaking, data catalogs are instruction manuals for how to understand and use data. A number of early AI data products, like Atlan AI and ThoughtSpot Sage, are efforts to make more intuitive interfaces for interacting with data. As all of these tools get better, the need for the instruction manual gets smaller—like how, despite everything it can do, an iPhone manual is a five page picture book.
I’m convinced that one day, Dominic Toretto won’t just drive a Dodge Charger, and James Bond won’t just wear an Omega; they’ll drive and wear whatever car and watch you just Googled.
Love Smitten Kitchen too! I was just thinking about making these again: https://smittenkitchen.com/2014/02/chocolate-hazelnut-linzer-hearts/
Nice analysis. My hope is that the created BS will bring us back to a more meaningful Internet somehow, just as it was initially intended.