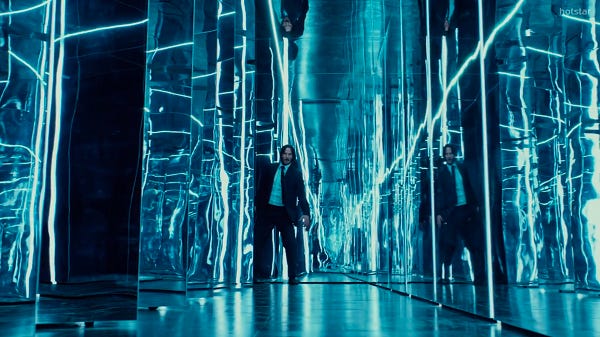
Imagine, once more, that you’re a venture capitalist. You invest in early-stage startups, the sort of companies that only have a handful of employees, a few wireframes and product prototypes, and a vision for how to make the world a better place. With no revenue metrics or customer feedback to base your investment decisions on, you bet on stories:1 Does the company have a unique idea, can the founders build it, and is there a market for what they’re selling?
You get an email from a startup requesting your time for a pitch meeting. You’ve heard through the grapevine that the company’s founders have been making the rounds among other VCs, and, despite being experienced in their field, they’ve been struggling to close an investment. But, being the founder-friendly and open-minded VC that you are, you decide to take the call.2
The pitch lands. Though you were skeptical of the initial concept, the story the company tells about its potential is compelling. You leave the meeting convinced of the founders’ brilliance for seeing a good idea in what seems like a bad one. You know you want to invest. The only question left in your mind is why other VCs didn’t feel the same way.
A day later, you get your answer. The company changed their pitch. Their proposed product never changed, but their story for why it’d be successful—the hole in the market it would fill, their clever plan for attracting customers, the viral loops that would drive its growth—was different in their prior meetings.
At first, you assume that they got their new direction from a grizzled industry veteran who suggested the strategic pivot. That’s fine, you think; they’re learning! Iterating! That’s the third step to the epiphany! But then, the other shoe drops. Nobody came up with the new plan—an AI did. After their initial struggles, the founders asked a chatbot for help: “Give me a compelling ten-point pitch for our startup, including details about why there is a market need for our product and how the business will grow.”
Their new narrative, in other words, was manifest out of thin air. It wasn’t based on feedback from a visionary expert. It wasn’t built on research, logic, reason, or a deep understanding of anything. It was conjured by a giant computer, a really good autocomplete algorithm, and a person looking for an argument to support a conclusion.
Do you still invest?
On one hand, the pitch is still the pitch. The plan is still the plan. Shouldn’t we evaluate the idea on its merits alone, regardless of its source?3 On the other hand, would we really not reconsider our choice? Does knowing that a pitch deck was based solely on the motivated reasoning of an AI really not undercut its apparent logic, illogical as that may seem?
The answer, I think, is we have to reassess. Not because the reasoning in the deck changes, but because we aren’t nearly as good at reasoning about the deck as we might think.
Data on trial
Some time ago, a long-simmering tension inside of Mode finally came to a head. For years, the leadership team knew that we had to choose between one of two potential directions to take the company. And for years, we'd punted on making the decision to “maintain optionality,”4 like a person straddling two boats that were slowly drifting apart. One spring, our flexibility reached a breaking point. We had to choose.
Since every prior effort to settle the issue had stalled, we decided to try something new: We staged a debate. A couple people were assigned to each option. They were instructed to make the most compelling case that they could for their side. Like a legal team fighting on behalf of a paying client, they weren’t looking for the truth; they were seeking a verdict. They had an argument to make, and a case to win. They were asked to use data to support their case, but the story they wanted to draw out of that data was predetermined.
This exercise, we thought, would surface all of the important issues we needed to consider. By pitting point against counterpoint, and compelling argument against incisive rebuttal, we’d rattle our way to a consensus. With such a wealth of evidence and analysis, our jury of sharp and well-informed execs would surely come to the right verdict.
In the end, we played ourselves. Because of various time constraints and other external factors, one team was able to make a lengthy and robust case in their favor, while the other had to rush theirs. There was a preponderance of evidence on one side of the scale, but its tilt was a function of the competing teams’ resources, not the evidentiary body that was out there in the world. And the jury’s decision—issued quickly in the expected direction—wasn’t the result of airtight logical reasoning about each case; it was a reaction to which argument was more available.
Foundational legitimacy
If we’re honest with ourselves, this is how most analysis works. When we read some argument or piece of analytical work—be it a proposal for a business strategy, a pitch for an investment, or an op-ed making a political point—we typically fancy ourselves as rational thinkers and impartial jurors. But we aren’t good at being either. As Randy Au called out in his most recent piece, sometimes "there are different hypotheses that all fit the data to a similar extent and there’s no way to tell which is the valid one.” Even trained analysts struggle to find the capital-T truth, and instead “fall into the trap of finding some initial findings that confirms a story,” with “no guarantee that any of the stories we weave out of data have any actual truthful basis!”5
Of course, we aren’t persuaded by every number or chart—we can detect obviously spurious correlations. But we aren’t particularly good at assessing when evidence is moderately weak or moderately strong. We’re plagued by biases, and when we evaluate the strength of argument, we rely on logically irrelevant context—what we want, what we think of the person making the point, the polish and energy with which they make it, how many other people agree with it—far more than we might think.
There is, however, one useful protection against us constantly being fooled by questionable reasoning: It's a lot harder to come up with decent arguments for something that's wrong than something that's right. Yes, we can torture data to make it say anything—but making it confess a lie takes a lot more effort than getting it to tell the truth. Reality, such that there’s such a thing in a dataset, is more available. We are protected from misleading conclusions and wild conspiracy theories not because we’re smarter than them, but because misleading conclusions and wild conspiracy theories are hard to create.
This difficulty—that, to make any kind of argument, we have to connect a bunch of dots in a seemingly reasonable way—gives most analysis a kind of foundational legitimacy: Someone had to figure it out. Someone had to weave a story that other people’s logical calculators, underpowered and imprecise as they are, would accept. If I want to make the case for why Mode should go down one path versus the other, or why “data scientist” is a bad job title, or why the 2017 Las Vegas shooting was an inside job, I have to figure out how to do that myself. I have to do the research, and construct the narrative, all my own. And the more wrong my conclusion, the harder it is to do that.
Or, at least, the harder it used to be.
Conclusions on demand
Last week, OpenAI released ChatGPT, the now-famous chatbot that can generate stories, poems, recipes, code, and millions of screenshots on Twitter.6 Backed by nearly seven trillion words of training data, ChatGPT can reply to nearly any query—including requests to make an argument that reaches a specific conclusion—with a remarkably cohesive and confident response. Want to defend the data scientist title? No problem. Want to create a conspiracy theory to argue that the Las Vegas shooting was actually carried out by the FBI? I can have one in seconds. Want to create another conspiracy theory that says it wasn’t the FBI, but was Coach K and the Duke basketball team? Just as easy.
None of these are real, in any sense. Even in examples when the underlying facts are true, there is no logical thread that ties one point to the next. They are spurious correlations, wrapped in plausible arguments, told with “high verbal intelligence.”
As Sarah Guo suggests, I’m not sure we’re ready for this.
In the 1970s, researchers at Stanford found the mere presence of an argument that supported a particular conclusion made people more likely to believe that conclusion was true, even when they were explicitly told the argument was made up. This suggests that, even in the absolute best-case scenarios—when we know some analysis was manufactured by a chatbot, with potentially no basis in fact or reason—we’ll struggle to evaluate the legitimacy of the story.
That’s bad enough on its own. How, then, can we possibly protect ourselves when the deception is less apparent? If we can’t unsee patterns that we know are illusionary, what hope do we have in evaluating the “seemingly-feasible” arguments that can now be generated for anything (both ones we ask of ChatGPT, and ones that others create)? Do we have any chance of making sense of that funhouse of mirrors?7
If ChatGPT starts incorporating data or citations into its answers, this gets even harder. Consider, for instance, the implications of this for even innocuous corporate examples. You go to a meeting to discuss a contentious decision. Each person brings a two-page narrative making a case for their desired outcome, complete with charts and statistics that seem to support their perspective. But nobody created their reports, or reasoned their way through what they say. Instead, they told a bot what outcome they wanted, and it dumped out a report full of facts, figures, and a plausible sounding story to explain it all.
Imagine the chaos that this introduces, not just in making decisions, but in understanding what’s even real. A few numbers and clever adjectives can completely change how we perceive the world around us. Today, it takes a rare combination of talent and expertise to persuade or, less generously, believably distort. But tomorrow, all of us may have access to an obedient research team that can justify whatever we want, with whatever bias we prefer.
The implications of that go far beyond the usual concerns about propaganda and misinformation (though those are real and significant), and could extend into even the most mundane of conversations. We use Google Maps to go places we know how to get to; we use Yelp to find restaurants we’ve already eaten at. When an acceptable rationalization for anything is a text message away, why would we not use it just as often?
For all the hand-wringing out there about the dangers of AI becoming too smart for us, it seems like we’re missing the much bigger problem: We’re not smart enough for AI.
The meta postscript
First, no, this article wasn’t written by ChatGPT; that joke is already overplayed. But I did ask it to rebut the argument that large language models threaten our ability to understand reality and make decisions. It echoed a point that others have made: Large language models surface more ideas. They open up new creative frontiers and expand the “marketplace of ideas.” We all just need to become more critical and capable thinkers.
Please. Does anyone believe that this will actually happen? We are lazy; we are illogical; we are biased. We will not collectively better ourselves in response to being slowly boiled by distortions of reason; we will sit in the soup until our brains melt out of our heads.
Take this very post as an example. I’m not an expert in AI, or in psychology, or any of the other subjects that I breezily Googled and cherry-picked links to.8 It’s likely that I committed various logical fallacies, misrepresented some ideas, got some wrong, and extrapolated too much from irreproducible and debunked studies. It’s also possible that the whole post is unremarkable, and common knowledge to anyone who’s spent more than a few days thinking about AI.
But do people have the time to consider all of that? (I know 18.8 percent of you don’t.9) The mere existence of the argument here, based on as little as it is, probably leaves some people in a different place than where they started. That’s not a huge problem today, because most people who don’t know about AI aren’t writing blog posts about AI. But tomorrow, there could be millions of posts just like this, disseminated by people with good intentions, by people with petty axes to grind, by legitimate bad actors, and by Discourse-addled teenagers who want to make a joke for the lolz. If you believe that people will be able to reason through that, I have an investment in a bridge-building startup to pitch you.
Who are we kidding, though; both early- and late-stage VCs invest a lot on vibes. Metrics and narratives and “rigorous diligence” is often theater, to rationalize the decision that they knew they were going to make after the first pitch.
“I’m not a VC, but an operator. I like to roll up my sleeves, get my hands dirty, do the work. I’m here to help, because I know firsthand the struggles of being a founder. I was basically one, once. What company? Oh, well, I meant I held leadership positions—erm, was senior product manager—at a Series B startup for two years, but, tomayto, tomahto.”
This phrase is a horrible trap.
This suggests an uncomfortable truth: Argumentative analyses—e.g., “we should change our pricing model” or “here’s why our campaign should focus on these voters in these counties”—are closer to conspiracy theories than scientific experiments. They create the illusion of connection and causality, but can never actually confirm it. As Randy says, the best we can do is create “a coherent whole that resists falling apart upon closer inspection.”
Silicon Valley hasn’t been this excited for a new product release since Pokémon Go.
Think about the last time you watched a murder mystery, like White Lotus or Knives Out. Every scene is designed to hint at potential motives, or tease us with just-visible coincidences. And off our brains go.
Look, this is benn dot substack dot com, not the New Yorker. If you want meticulously-researched and reported stories, read Ronan Farrow. Come here for a conversation with a grumpy guy at a bar who doesn’t usually drink caffeine, is three espresso martinis in, and has had a bad day.
If you haven’t already, fill out the reader survey! The 58th respondent to this week’s version wins a Logitech C920S HD Pro Webcam!
I can't help but think of the Internet itself as an analogy for AI.
Yes, it brought on plenty of things the world would be a better place without, but it also brought so many wonderful things like working from home :)
We'll just have to adapt and accept that it's becoming easier and easier to influence the masses.
Perhaps there is much to worry about; perhaps not.
The more there is to doubt, the more I suspect (hope) people will practice active skepticism about every single thing they consume, no matter the source. When it becomes obvious that anything can be manufactured and manipulated (it's not like AI was needed for this), people will need to adapt in order to avoid harm.
So I'm banking on the rise of AI to lead to a healthy rise in human skepticism and thoughtfulness. Or Skynet. 50/50.