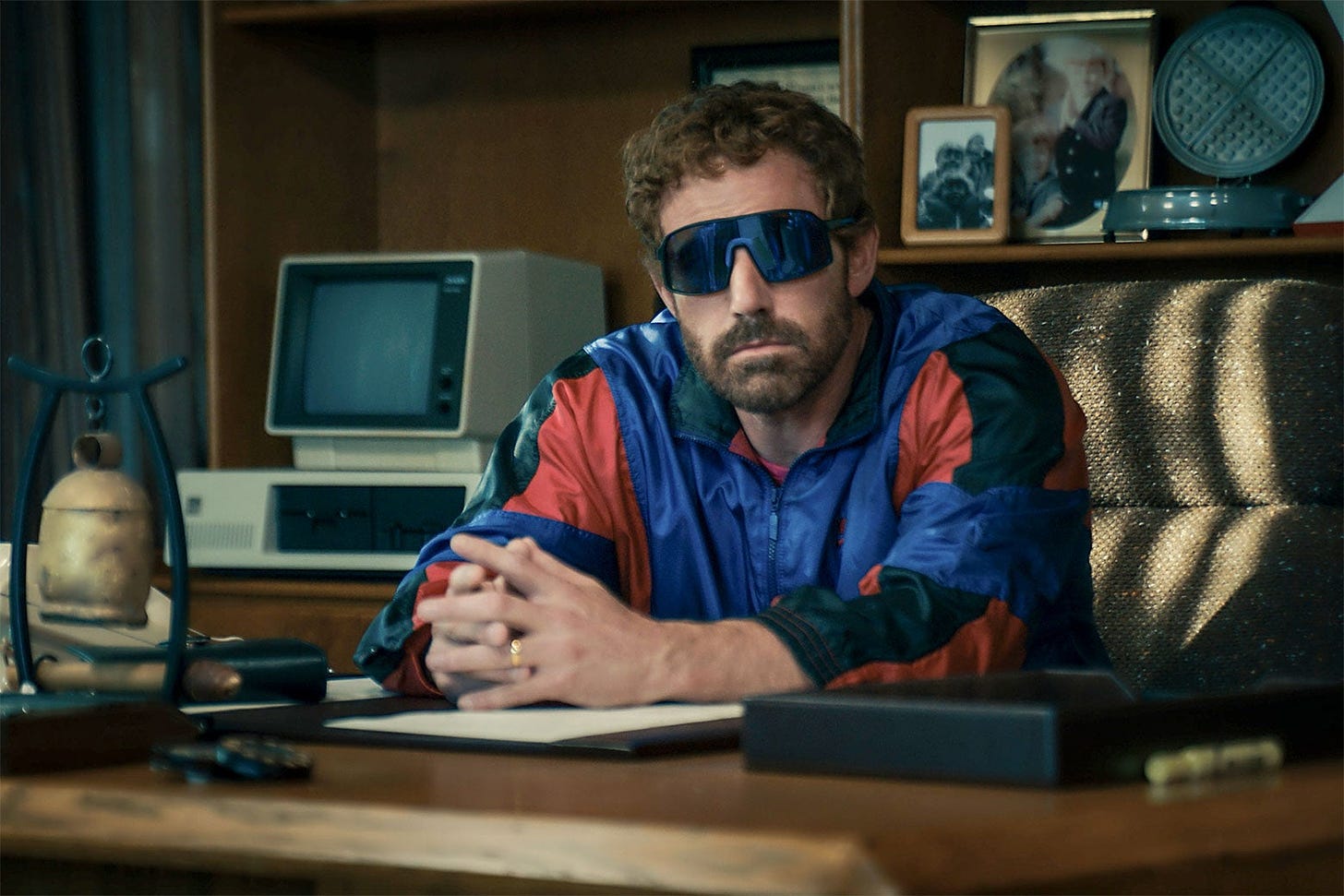
If you’re reading this, there’s a decent chance that you’re here because of a post I wrote in 2021 called “The missing piece of the modern data stack.” To the degree that a gossip blog about startup middleware has “hits,” that article was my biggest one: It doubled the subscriber base (into triple figures!), has directly accounted for more signups than any other article, and is the third-most viewed thing on this Substack, despite fewer than 100 people getting the original email. If you could trace the series of events that led you here—from someone reading something to sharing something to someone else reading something to sharing something else, to it eventually leading you to this spot, right now—that post would probably be the first domino.
It’s also the post, and perhaps the only post, that has moved the world in some detectable way. The metrics layer—the name I gave the part of the modern data stack that I said was missing—has been adopted by a handful of content marketing teams. It was born; it was explained; it was debated; it died. Some startups tried to build it; Google spun “a standalone metrics layer” out of Looker. For a moment, it became A Thing.1
It was also a bad idea?
I mean, I don’t know that, not for sure, not yet. But the trajectory isn’t great. By the end of 2021, at least six companies were building a product that could reasonably be called a metrics layer. Two pivoted, one got acquired, and one stalled. Two are still growing—Cube raised some money in June, and dbt still sells their semantic layer—but neither have become anything close to a market standard. Google has gone silent about their spinoff. In 2022, the industry was chasing the idea; now, after some false starts and disappointing v1’s, it’s slowly backing away from it.
Perhaps this all changes. Perhaps it just takes a few iterations; perhaps things standardize slowly, and then all at once. I wouldn’t bet on it—I already tried that, in a couple ways—but you never know.
I say all of this to make two pretty obvious points. First, bad ideas sometimes sound like good ideas, and second, until you try it, it’s really hard to tell the difference. We can all debate the viability of some new idea on Twitter forever; we can all come up with some clever reason why our side is right; someone could even “win” the argument by saying something that sounds more clever than what everyone else said. But until someone goes out and tries the thing, we haven’t figured anything out yet. A compelling rhetorical argument isn’t the same thing as the truth. The only real truth is what happens.
But what if that argument is made with logic and reason? What if it’s made with data? We’ve talked about this before: These are still just arguments, and logic, reason, and data are, outside of mathematical proofs, still just rhetorical devices. Filling that blog post about metrics layers with charts wouldn’t have made it any more true; it would’ve just made the argument more compelling, and harder to disagree with.
Of course, there is some subtlety here. If we accept that there is some Objective Truth out there—that the metrics layer doesn’t work, or, to choose a random headline from the New York Times this morning, that interest rate cuts won’t fix a global housing affordability crisis—it’s probably easier to make both logical and quantitative arguments in favor of that truth than it is to make arguments in support of the opposite. That’s why analysis works, to the extent that it does: Not because it finds the truth, but because finding a sound argument for something that is true is easier than finding a sound argument for something that is false.2
Anyway, a month ago, a former brand director at Nike published a rant about how the company screwed up their brand. The post accused Nike of making a long list of strategic mistakes: They tilted too far towards direct-to-consumer digital sales; they favored brand design over brand communication;3 they decided to “eliminate categories from the organization” and to rely less on their wholesales business; they mismanaged their supply chain; they focused too much on their loyalty programs. They did a lot wrong, and honestly, if you don’t know how global apparel businesses work (which I do not), it was a little hard to follow.4
But the internet quickly collapsed the post into a single viral headline: Nike’s original sin was trying to be “data-driven.” From the post:
A few months later, after his first tour around the Nike world, the CEO announced – via email – his decisions: … Nike will change its marketing model, centralizing it and making it data driven and digitally led. …
Because of that, Nike invested a material amount of dollars (billions) into something that was less effective but easier to be measured vs something that was more effective but less easy to be measured.
This part of the post broke through the LinkedIn firewall and into the general discourse.5 We must be more careful with how we use data, people said; data is not reality; the map is not the territory. Others had harsher criticism: This was “fixed-mindset thinking;” Nike’s “incestuous strategy” spun them into a “data-driven death spiral.”
Which, ok; the first set of points seem true enough; the second set of points probably aren’t wrong, though they feel like an over-extrapolation of the original post. But even if we accept all of this as true—that Nike did what the person on LinkedIn said, and it failed for the reasons that the internet said—it still leaves a glaring question unanswered: Why didn’t they know better? Rough truisms like “data has blind spots” were surely as well understood by lifelong Nike veterans as they are by internet bloggers and LinkedIn thought leaders. If it is so easy to criticize Nike’s strategy from a distance, why was it so hard to resist it from up close?6
The problem, I imagine, isn’t data, exactly; it’s culture. Even though most people and companies aren’t data-driven in the canonical sense—they aren’t making decisions by responding to some flickering Bloomberg terminal of charts and numbers—most people are at least data-pilled. We like Moneyball. We like the old OkCupid blog. We thought Nate Silver was a witch. We “trust science;” science is objective; science uses data; data is objective; in God we trust, all others must bring data. Though we understand that data has limits, twenty years of cargo culting about Google and Netflix and Renaissance and the Oakland A’s put it on an argumentative pedestal. If you want something, you can 1) just say what you want, 2) say it, but loudly and often, 3), say it in some clever way, or 4) say it with a graph. Though there’s not that much difference between those things, modern society strongly prefers the fourth version.
This, I think, creates a kind of vibes-based meta-bias. Rather than favoring the highest-paid person’s opinion, we favor the SWAN (Suggestion With A lot of Numbers), almost regardless of what those numbers say. The effect of this isn’t to mindlessly optimize the stuff that we can measure, but to assign a certain amount of truth to arguments made with measurements. I doubt that Nike focused on Nike.com memberships and forgot about “brand magic” because they could easily measure the first one and not the second one. I do suspect, however, that the team that was working on Nike.com memberships could make a lot of compelling arguments about corporate strategy that the team working on brand magic could not. When both teams are pitching their ideas, they are pitching things that probably sound like good ideas, but might be bad ideas. But if the memberships team can make their pitch with numbers and forecasts and statistics, it sounds less like an opinion and more like a fact.
Put differently, doing quantitative analysis feels like figuring something out. It feels like a shortcut to the end; it reveals “insights;” it is a way to know something, or at least get reasonably close to knowing something, without doing something. But it is not. As Nikita Baer put it in a recent podcast, the only real way to figure anything out is to get punched in the face:
The most important thing that I often instruct teams to do is to develop a reproducible testing process, and that will actually influence the probability of your success more than anything. It's so unpredictable whether a consumer product idea will work. If you actually focus more on your process for taking many shots at bat, that's what actually reduces the risk more than anything.
Or, more succinctly, from Cedric Chin: “There is no ex-ante strategy for finding a secret beyond “fuck around and find out.’”
Data is useful for finding out by being useful in measuring interventions—the experiments, the building of the metrics layer, the new organizational structure at Nike. It is useful as a step in the scientific method, not a replacement for it.
To paraphrase a coworker, the opposite of a debate-driven culture is not a data-driven culture. They are actually the same thing, just with debaters wielding different weapons. Instead, the opposite of a debate-driven culture is—appropriately—a do-stuff-culture.
To be clear, a lot of this stuff was in the works before that post got published, and other people—like Alana Goyal at Basecase Capital—have just as much claim to this idea as I do.
I’m honestly not sure if this is a radical belief, or very obvious? Or just dumb? Either way, to me, the interesting thought experiment is to imagine a world where you could generate a logically sound argument for anything. Surely there is some arrangement of words out that would make an seemingly airtight argument about why the metrics layer is a catastrophically bad idea, and surely there is a tortured set of economic data that seems to prove that interest rate cuts will fix a global housing affordability crisis. How would we deal with that? If there is a good case for every idea, it seems like our ability to reason would sort of break down at that point. (“If you think about it,” the college sophomore says after a huge bong rip, “wHaT iS tRuTh AnYwAy?”)
Guess which one the author works on!
Should a company that sells $50 billion worth of clothes every year have a centralized marketing organization or a matrixed one (whatever that means)? Should they eliminate categories from the organization (whatever that means)? Should they focus on “‘mono-brand’ distribution” (whatever that means)? I have no idea? But the stock is down, so they’re obviously morons.
(whatever that means)
Again, I’m assuming all of what was said about Nike is true, so that I can barf out more internet blogs and LinkedIn thought leadership. I have no idea if any of it is actually true. (“But, like, woah, can anything really be true, man?”)
Hey Benn, awesome article as always! Not sure if you got my email - I have a draft of an article that I want to publish on the Medium publication Towards Data Science on how to fine-tune a LLM by using your content to teach a LLM to write like you. But, I need your permission. It seems like you're pretty responsive through comments so I thought this would be a good way to reach you :) anyways, I emailed you from mathewnxwang@gmail.com if you want to check it out or if you're already down feel free to just reply to this.
I think (especially for tech companies) the data driven approach is starting to just feel more comfortable. It is what you are "supposed to do." If you are in tech/data it is easy to sit down at your keyboard and query a database looking for something to support your theory. (Or ask someone else to do this for you). It feels harder to talk to a bunch of customers, record the results, and study and make sense of what they said... AND that also feels less sophisticated like something "anybody" could do.
People want to see predictive models and fancy charts not tabulated customer data manually and try to find signal in a bunch of noise - when a signal might not even exist.