Honeydews are the most enigmatic melons.
The average honeydew—the kind you get in a continental breakfast at a Hampton Inn, alongside a few stale bagels and an empty tray where reheated sausage once was—is always slightly too firm and slightly too bitter, as if each piece has a bit too much rind. The average cantaloupe, the honeydew’s inalienable partner in every cafeteria fruit cup you’ve ever had, is much better: We eat five times more of it, even though it costs fifty percent more.
But everyone once in a while, you luck upon that perfect honeydew—sweet, cold, just the right amount of juicy tang, a beautiful refreshing green. That honeydew isn’t just better than every other honeydew; it’s also better than even the best cantaloupe or watermelon.1 That honeydew is why the disappointment of the last piece doesn’t deter us from trying the next. That honeydew is why we don’t forsake all honeydew for the more reliable cantaloupe. That honeydew is why we keep eating honeydew.
We analysts, I fear, are honeydews.
Two weeks ago, I wrote that analytics isn’t a technical field. Our job as analysts is to help companies make good decisions, and that requires curiosity and critical thinking, not an advanced degree in computer science.
For the most part, people agreed, and were exasperated by the effect of this mislabeling. By coloring analytics as a technical function, our employers discount the importance of analysis itself. The decisions we support are more valuable than the technologies we build, but organizations aren’t structured to recognize that; social status and the power dynamics of Silicon Valley compel us to overvalue engineering skills; we’re kingmakers, and people don’t see it. The collective sentiment was well summarized by an email I got from an experienced practitioner: “Senior leadership hasn't fully internalized the shift from ‘reporting’ to ‘analysis’. If you think that an analyst’s job is just dumping numbers into Excel...of course you're not going to want to pay top-tier salaries for real analysis.”
In other words, analytics teams are victims of the soft bigotry of low expectations. Many companies expect data teams to churn out reports and reconcile meaningless wiggles in dashboards—that is, until they work with a team that’s proactively influencing company strategy. Execs, department leaders, and perhaps even us analysts don’t realize what we’re missing until we see it for ourselves. We’re constrained, both financially and hierarchically, by a misunderstanding of what we do.2
But underneath this reassuring affirmation that our potential is crackling just below the surface, waiting to be set free, an uncomfortable question kept nagging at me: Are analysts actually misunderstood and undervalued? Or—to touch the rail we rarely touch—are we just not worth that much?
There’s no denying that analytics can be valuable. Our job is to create the quantitative and “intellectual underpinning behind a company’s most important decisions.” Help a company avert disaster or pivot into a $20 billion printing press, and you're worth your weight in gold.3 Even the more modest wins—uncovering a tweak for improving checkout rates, or putting together a great market analysis that closes a funding round—are worth as much as the normal contributions of any engineer.
When we talk about the value of analytics, it's easy to point to these examples. It's easy to say, as I said two weeks ago, that these are the hard things we're paid to do. But as tempting as it is to define our worth by the superlative among us, others will measure us by our average representatives. And the accomplishments of the average analyst—or even the average accomplishments of the best analyst—can be underwhelming.
Because there's a corollary to our job being the hard things: They’re hard to be good at. To produce valuable analytical results, you have to be quantitatively sharp, a good deductive thinker, have a deep understanding of the business domain you work in, be a crisp and fluid communicator, be persuasive without being pushy, and get a couple lucky breaks when looking for needles of insight in haystacks of data. It’s a lot to ask. And it’s possible—or likely, even—that, for most of us, it’s too much to ask. Given the difficulty of problems we’re asked to solve and the long list of skills needed to solve them, answering routine questions may be the most useful thing we can repeatedly accomplish. The average engineer, by contrast, can regularly deliver more valuable results—the consistent cantaloupe to our erratic honeydew.
Importantly, this isn’t to say we're bad at our jobs, or that the analytics industry is full of talentless hacks while engineering departments are stocked with intellectual thoroughbreds. The problem is the role itself, and the steepness of the hill that it sits on. Sometimes, even for Sherlock Holmes, the case is too muddy and the trail is too thin.
Indirectly and fitfully, we often concede this ourselves. Behind every weary joke about building too many unused dashboards is an acknowledgement that we spend a lot of time building unused dashboards. Implicit in every complaint that our work doesn’t change stakeholders’ minds is an admission that our work frequently doesn’t change stakeholders’ minds. We talk a lot more about our potential, always just over the horizon, than our present.
The usual suspects
The two most common explanations for these shortcomings are that we’re shackled by unimaginative bosses and immature tooling. On the former point, analytics teams are definitely held back by organizational muck. But we’ve been fighting this battle for a while, and company leaders aren’t dumb. If they’re still not seeing something, we might have less to show than we think.
Trevor Fox made the case for the latter point in a Locally Optimistic thread on this subject, arguing that “analysts'...capacity outpaces the tooling to support it.” Broadly speaking, the ambition of the modern data stack is to close this gap. Rather than building or maintaining infrastructure, data teams should, as Erik Bernhardsson said in the same conversation, aspire for “100% of code written to be business logic.”
I’m supportive of this goal. It’s also probably achievable: Over the last fifteen years, cloud computing platforms and application frameworks like Rails did an analogous thing for software development. Computer engineers are now much more likely to be software developers working on application logic than closer-to-the-metal programmers who build foundational infrastructure.
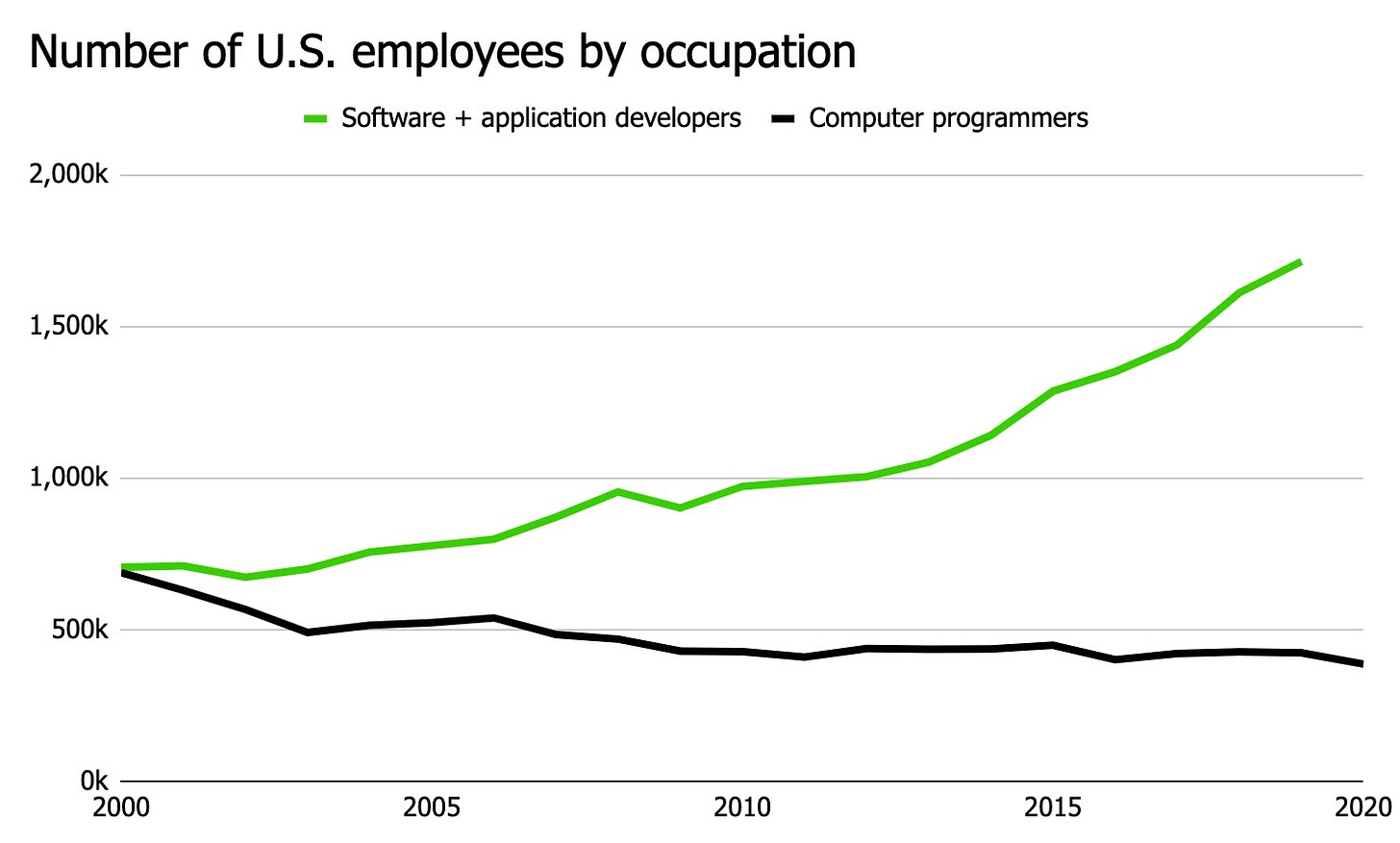
But better frameworks may not be enough. After all, interpreting data, even data that’s neatly and painlessly served up to us, is what’s truly hard. While having more time to do that, and a cleaner environment to do it in, would probably make us better at it, most pieces of the modern data stack are indirect solutions.
Moreover, we’ve been waiting for better tooling for a while. At some point, we’ve got to make do with what we have. “A poor craftsman,” as they say.
Mirror, mirror, on the wall
That leaves us with a third discomforting explanation for why analytics isn’t as valued as we’d like it to be: The problem is us, and we’re not as good at this very hard problem as we think we are.
I’m not convinced that’s true. It is, after all, difficult to get a man to understand something when his salary—and his equity in an analytics company—depends on his not understanding it. But for the sake of finding ways to be better at what we do, what if it is true? What if our struggles aren’t those of a misunderstood, poorly equipped team, but of a healthy, well-supported one, trying its best? What would we do then?
To extend the honeydew analogy, I can think of two options: Better selection, and better cultivation.
On selection, we can recruit and hire people who are better suited for the job. Our current technical sieve provides the wrong mix of people, in two ways: It's overly porous for people who excel technically but lack many of the other necessary skills, and overly narrow for people from non-technical backgrounds who are creative thinkers and persuasive communicators.
At a minimum, we should be more direct about hiring for the skills people actually need. Junior data scientists, as Vicki Boykis recently noted, are sold roles that don’t exist. To paraphrase Maya Angelou, when we advertise a job and people show us that they’re interested in the responsibilities we pitched to them, we should believe them. Instead of scoffing at junior folks’ naiveté—”silly child, you thought you were going to be tuning models, fix these eight revenue dashboards instead”—as though their disappointment is necessary hazing for becoming a bitter senior analyst, we should swallow our pride, describe the job honestly, interview for those skills,4 and find people who are excited to do the job we’ll be asking them to do.
Beyond improving how we select analysts, we can also better cultivate them. Tristan Handy alluded to this problem in his (very kind) response to my post: We all seem to be at a collective loss about how to train analysts to do the squishier parts of their jobs. Hopeful analysts can learn computer science and statistics in school; numerous bootcamps and online tutorials offer crash courses in SQL, Python, and web development fundamentals; graduate courses in analytics teach textbook business principles and applied statistics through manufactured capstone projects. But want to practice problem solving that will make you a better analyst? Even Reddit can’t help you.
At best, this lack of training leaves most new analysts unprepared for the realities of their job. At worst, they’re unaware of what that job even is. This gap is apparent in our “senior shibboleths:” It shouldn’t take several years of experience and frustration for aspiring data scientists to realize that SQL will probably solve more problems than AI. But it often does, and that’s our failing, not theirs.
As much as we invest in analytical technology—from the billions that investors put into data vendors to the billions that companies spend purchasing those tools—it's worth considering if we're investing enough in the people who make those tools useful. Without them, all our technology is just expensive servers and websites, churning through busywork. The best analysts have shown us that these investments can be worth it. But the rest of us have to do more than celebrate those superstars and claim their abilities as our own, if only our confused managers could see it. Perhaps they are seeing it, and we’re the ones who are confused. And what they see is a melon with more potential than juice, the inconsistent addition to the company fruit bowl, in need of better culling and more cultivation to live up to its promise.
In fairness, I’ve never tried a $311 Sentinel watermelon.
This problem isn’t unique to analytics. In Silicon Valley, a lot of companies treat HR as corporate hall monitors who get in the way of growth, or crushing it, or “jokes.” Work with a great leader, however, and you quickly realize that HR isn’t organizational tar, but an invaluable strategic asset.
For those wondering—and God help you if you were—181 pounds of gold is worth $5.2 million dollars. Major tech companies make about $750,000 per employee, and people work at these companies for about 3 years. So, given the choice between an analyst and a statue of that analyst made out of solid gold, take the statue.
Few things set the tone for a role more than an interview. Ask brain teasers, and people will think their job is to win Mensa contests; bring people onsite for a day of work, and they’ll see the job as working on open-ended problems with others; mindlessly chat them up, and they’ll think (and look, and act, and be) exactly like you and your college drinking buddies.