Data arithmetic
Science is for suckers. Plus, influencers giveth, and influencers taketh away.
Of all the games you can play in a casino, roulette is the least mathematically interesting. In blackjack, there are strategies for how to play; because most casinos deal multiple games from a single shuffle, your odds can also vary by hand.1 In craps, each roll is independent, the odds are at least somewhat complicated to calculate.
Roulette is one giant dice roll. Every bet is mathematically identical—you bet on some number of pockets on the wheel; your odds of winning are the number of pockets you bet on divided by the total number of pockets. That’s it; that’s the whole game. Wikipedia explains it all with fourth grade math.
A former analytics coworker of mine2 was recently in Las Vegas, and noticed that the roulette tables are now equipped with dashboards that show historical results from previous spins. There were tables, distribution charts, and callouts for which numbers were hot and cold. People were studying them, he said, like day traders who look at the random twitches on a stock chart and see alpha. “On this table,” they must’ve been saying to each other, “there’s a congestion area from numbers 10 through 14, and the 6’s double top is establishing a clear resistance band, so I think everything below 18 is a bull trap. The measured moves of numbers 30 to 36 are already near the support level and starting to test the top, so I’m betting hard there.”
Hahaha. Fools. How ridiculous of them, these ignorant wretches, intoxicated by a few charts and blinking lights, drunk and dizzy from staring into an endless abyss of meaningless numbers.
...I say, as I return to my day job of churning out glittery dashboards, of squinting at oscillating trend lines, and of ad-libbing my own methods of technical analysis—“we’re below our goal but if draw a regression line through a few arbitrary points, and adjust for the holidays, by ignoring them, and extrapolate that trend forward by three months, then we can see…well, we’re still behind, but we think it will turn around, because that which is measured, improves, right?”
Cedric Chin has seen enough of this sort of astrology:
There are a gajillion articles about 'the modern data stack' and 'data warehouse good' and 'how to do data modeling', and not a single one about what to do when you look at a chart.
When you open a business dashboard in your company, do you feel a little confused? Like you don’t know what to do? I mean, look at this chart [he shows a wiggly line, with no clear pattern]. What can you conclude from it? Is the metric doing well? Is it going up overall? Is it business as usual? Or are things getting worse? Should you be worried?
If you’re like most people — myself included — you would not know how to read actual charts of real world operational data. You would open up Google Analytics and think … “so what?”.
Chin has been thinking and writing about this problem for a while. His solution to our confusion, forged in the operational trenches of companies like Amazon and Toyota, is a six-step process:
Look at how things are going.
Figure out when things were going better or worse than usual.
Hypothesize why that might’ve happened.
Do more of the potentially good things and less of the potentially bad things.
See if things get better again.
Learn how things work.
On one hand, yes, obviously? Of course this is what we should do. We do this all the time. We listen to a song by a new band;3 we like it more than the average band; we listen to another song; we learn that we like that band. We day trade crypto; we lose money; we try it again; we learn that day trading crypto is a control factor that influences the balance of our bank account. We email a bunch of sales prospects about our product; we get more positive responses than usual; we try that same email again and don’t get more positive responses; we learn that we probably just got lucky the first time.4 None of this is rocket science. It’s barely even science.
On the other hand, there’s something profound about Chin’s post. Despite having read hundreds of Medium articles and VC blogs about how about which product adoption metrics are best, or how to understand user cohorts, or how to benchmark your company’s KPIs, it was Chin’s post, and only Chin’s post, that inspired me to immediately dump a bunch of Substack metrics into a spreadsheet and see they taught me.5 Why, despite the underlying theory being simple, obvious, and something I already do informally every day, did Chin’s articulation of it land so differently?
One answer is packaging—it’s an engaging read; he prescribes a way to find anomalies, called XmR charts, that have a satisfying visual aesthetic; the social proof from Amazon, and from the depth of Chin’s own research, is hard to ignore. But none of those things are that unique. Every data conference is full of glossy presentations about how some amazing company built itself, and we often start forgetting those talks before they’re even over.
The better answer, I think, is that Chin’s post gives us permission to make this simple. When we look across a sea of data, it's easy to be overwhelmed by the difficulty and depth of it all. It's easy to assume that there are so many complications; so much confounding; so many numbers; so little time. When working in this sort of environment, we often play up to the complexity of our competition—by using candlestick charts, or fancy math, or formal theories of causal inference, or our time-honored tradition of smugly saying, “well, you see, it depends.”
Chin tells us to throw all of that out. He’s studied this more than we have, and has talked to people who made this work better than we did. And he says it’s okay to draw some trend lines, to kinda eyeball some error bars, and to not fret too much about the details. The power of this instruction isn’t in what it tells us to do—most of us are already pretty accustomed to looking at dashboards and trying to figure out why it went up and down—but in what it tells us not to do. Don’t try to solve your problems in a novel way, he says; try just drawing these charts first. Don’t worry about those wiggles, unless they persist for too long or get too severe. Don’t worry about the precise methods for detecting anomalies; use this simple formula.6
As an analyst, an executive, a day trader, or even a blogger starting at a few Substack dashboards, every chart is a temptation—surely, you think, if you look at it at just the right angle, it will reveal its secrets. This is why casinos, which control every input on their gambling floors, put dashboards on roulette wheels—not to inform us, but to bait our natural inclinations to see patterns in every chart.
Chin reminds us of the danger of this approach. When you investigate everything, you find nothing; when you find nothing, you doubt your methods; and when you doubt your methods, you flail:
You discern no clear pattern; you chalk it up to a “maaaybe?” and move on to the next idea. You don’t get any feedback on your moves. You’re like a blind archer, shooting arrows into the dark.
But we already know how to aim: Look for variation, ask what caused it, repeat. We don’t need to teach ourselves how to do this; we just need to be reminded—as Chin is reminding us, now—that those simple steps are enough.
ALD
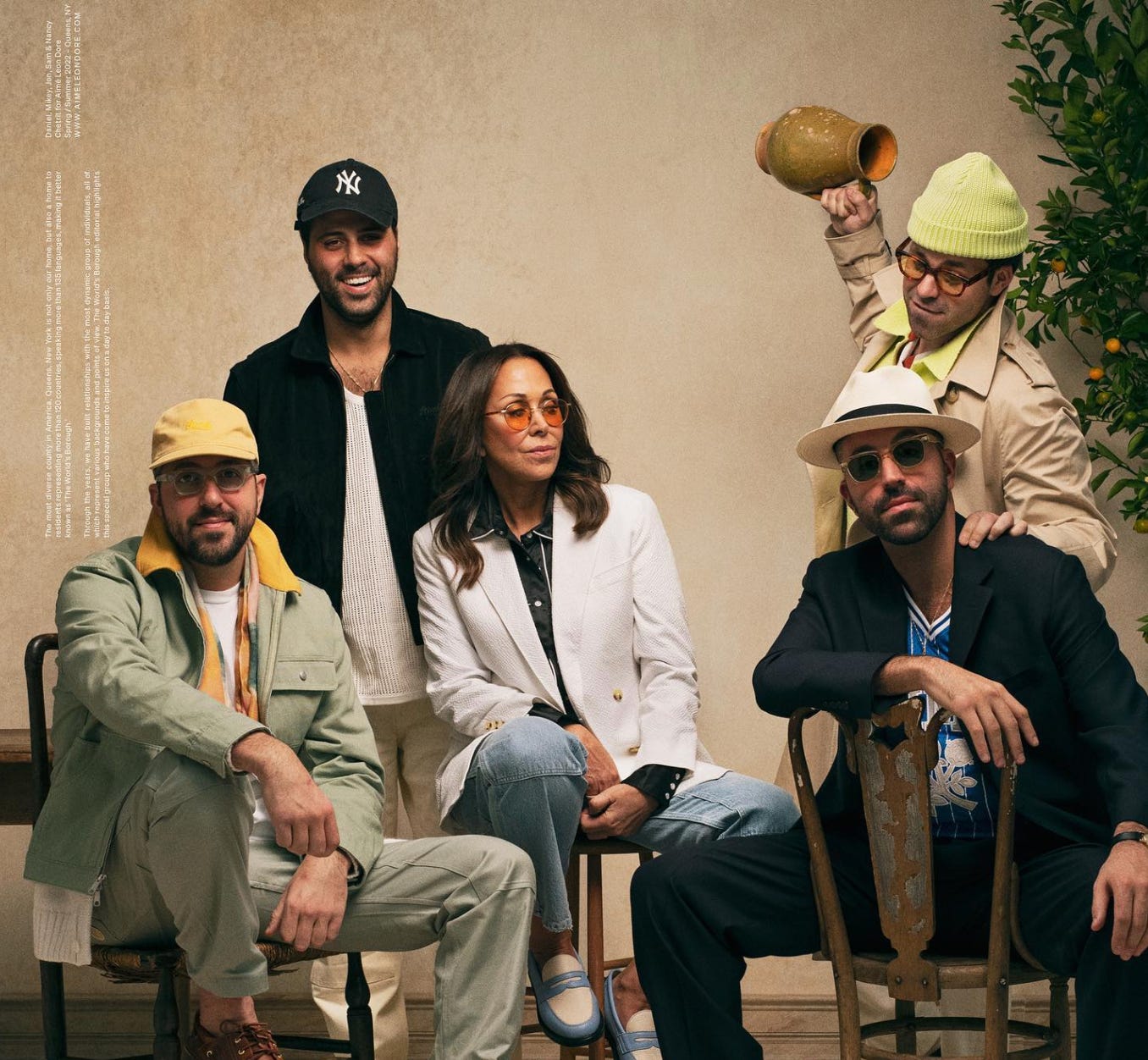
Aimé Leon Dore, commonly known as ALD, is a popular men’s fashion brand based in New York City. Like most lifestyle boutiques, it’s popular for two reasons: Because people like its aesthetic, and because people like the people who like its aesthetic. From a profile of the brand in the New York Times, “the ALD experience extends beyond clothes into…what worlds wearing those clothes will associate you with.”
Today, that world is Queens; that aesthetic is vintage Ralph Lauren and classic hip-hop and basketball; those people are George Costanza and Nas. According to ALD’s About Us page, you can learn more about ALD by listening to Nas’ Illmatic; according to Nas, he wears Aime Leon Dior New Balance when he’s steppin’.
Greg Gutfeld is a 59-year old white Fox News host. He is, in his own words, relentlessly pro-business. On his Fox show, he’s interviewed Vivek Ramaswamy, Piers Morgan, and Dennis Miller. He wrote a book declaring that the “hipster elite” aren’t cool, arguing that “the real American ideal of cool” is “building businesses, protecting freedom at home and abroad, taking responsibility for your actions, and leaving other people alone.” He’s navigating a midlife crisis by wearing colorful sneakers.
This month, he also started wearing ALD.
So that’s awkward. ALD, and to some degree every lifestyle brand, is valuable by association. It’s cool because its customers are cool. What do you do, when you spend years building a brand out of the multicultural and colorful flair of the “world’s borough,” and suddenly every Republican dad’s favorite variety show host starts wearing your sweaters on national television? What do you do if his fans—fans like Thaddeus, who “will never like hip hop”—start buying your polos?
Can ALD pay Gutfeld not to wear their clothes? If they did, could other Fox News hosts extort their own anti-sponsorships? Imagine—Brian Kilmeade in Kith; Neil Cavuto in WTAPS; Newt Gingrich in BAPE. If oil companies can get carbon credits for not drilling oil wells, can society’s undesirables—white nationalists with eleven million Twitter followers, hated musicians,7 the Sackler family, millennials—get paid to not stain cool things with their poisonous endorsements? Is this how we finally break the Gen Z obsession with Stanley, not by talking about how dangerous they are, but by talking about how much we love them too?8 The Five loves to complain about the creative power of Taylor Swift’s endorsements, but has the The Five thought about the destructive power of theirs?
Or would that only make these brands stronger? Could ALD embrace Gutfeld, not as a sponsor, or an anti-sponsor, but as a kind of double-agent sponsor? They made something so cool he couldn’t resist it, and their commitment to that coolness—proven by their willingness to sacrifice the brand rather than pay Gutfeld a dime—only makes them cooler. By attempting to ransom money out of ALD, Gutfeld would only make them more of a hero. By trying to strike them down, Gutfeld would only make them more powerful.
I mean, on one hand, who knows, who cares, how did we even get here. On the other hand, the world does kind of work like this now? We buy tons of stuff because influencers tell us to. People have careening opinions about the NFL, vaccines, Tesla, and Bud Light because of who’s in their ads and who promotes them. I don’t want to buy a business class seat on Twitter, not because of what it costs, but because who’s charging me for it. If you’re an influencer—or whatever Greg Gutfeld is—it seems short-sighted to only ask to be paid for the things you do. You can only do so many things! Much better to get paid for the things you don’t do.
This is how card counting works. Basically, players play multiple hands, keep track of what cards are left in the deck, and adjust their bets accordingly.
Well, I don’t; I just obsessively refresh Griff’s Spotify page waiting for her to drop her next single. But people tell me there are other bands out there.
So look, I’m fully behind Colin Cowherd here (which are words I never thought I’d say), but I did just get this cold sales email and my resolve is wavering:
SUBJ: will Taylor Swift make it to the Super Bowl from Tokyo? [either capitalize “will,” or commit to the lower-case reply guy bit and get rid of all the capital letters. I’m already bothered.]
Hey Benn - Are you up for another round of friendly competition? [Another? Have we done this before?]
Remember our last wager? [no] It was such a hit [it was?], we're back at it [back at what?] for the Super Bowl – but with a “Taylor's Version” twist! [Oh haha, very good, I see what you did there, how very creative.]
For everyone with a Swiftie in their life or for those who just love a good surprise, here's the deal: Will Taylor Swift jet from her Tokyo concert to the Super Bowl in Vegas? [What? Yes, sources already say yes] Just send in your guess! [If you read this, this does not count as my guess, I am not guessing.]
Yes – She'll make a surprise appearance! [A surprise? Darren Rovell tweeted about it before the game against the Ravens was even over. Both United and American Airlines have gimmick “1989” flights to Las Vegas. Who could possibly be surprised?]
No – She won't be there. [But she will be there? I guess she could take a Boeing?]
If you guess right, choose between a pair of Apple AirPod Pros [Ok I do want those though] or a Sonos Roam SL – perfect for jamming to Taylor's hits [I mean, she does have some bangers]. And if she doesn't, or you guessed otherwise, enjoy a $50 DoorDash gift card will be yours to enjoy a tasty treat on us. [Is this some sort of riddle? If I guess yes and she goes, I will have guessed right and win the AirPods. If I guess yes and she doesn’t, I’ll be wrong and she won’t have gone, so I’ll get the DoorDash gift card. If I guess no and she goes, I’ll be wrong and I think I’ll also win the DoorDash gift card? But if guess no and she doesn’t go, I’m right? But she didn’t go, so I get the gift card?] It's a win-win! [I’m honestly confused about what winning means at this point.]
So, what do you say, Benn? Ready to make your guess? Simply reply to this email with your prediction and schedule a time to chat this week or next. [Taylor Swift generated $331 million in brand value for the Chiefs, but how much revenue has she generated for remote network access IT solutions?]
I made a chart! Of new blog subscribers! Which, two thoughts:
Even for very prescriptive methods like this, there’s still an entire garden of forking analytical paths. Over what window do you create your average and moving ranges? And should it be rolling, or, something like a quarterly or annual step function? Or constant for the entire time period? I’m sure there are some recommendations here, though I couldn’t find any. In any case, it didn’t really matter here, and the conclusions were basically the same: Something changed, and for the worse, in the fall of 2022.
What was that thing? Did the articles get bad? I mean, probably. Did I hit a niche data blog’s invisible asymptote? Also very possible. But the timing also corresponds almost exactly to when Elon Musk bought Twitter, and throttled the reach of people who don’t pay his ransom. Which I am loath to do, but—and this is exactly Chin’s point about why XmR charts work—I’m now really curious to pull the lever and see what happens. Ugh.
As Chin points out, his specific formula, the XmR chart, isn’t actually necessary. Over time, other methods may make sense. But XmR charts are good starting points, because they generally work, because they’re easy to build and understand, and because they’re a good way to break people’s habits of looking at metrics as something to optimize, and instead think of them as a way to figure out how controllable inputs affect desired outputs.
Or has Scott Stapp has pulled a Keanu Reeves, and horseshoed around from being so uncool that he’s now cool?
When I was in middle school—in North Carolina, where dipping tobacco was very popular—my mom told me that I could dip if I wanted to, but if I did, she would too.
Edward Deming and his system of profound knowledge come to mind here. The realization that many dashboards simply result in what Deming calls “tampering” by management. And unfortunately the unexplained wiggles are some of the most tempting data points for this…
I have thought a lot about simple metrics vs flashy data insights recently.
The data/dashboards that make a difference in my personal life on a day to day basis are never “insights.” Here is a short list.
1) fuel gauge on my car - I get gas when it’s low
2) apple watch 3 rings - try to go on a walk when my exercise ring is low
3) scale - try to adjust my exercise to calorie ratio when the number gets higher
No amazing insights from data - just numbers with clear actions to take to change them.
As has been mentioned - accountants have this stuff nailed down pretty well. Not sure why it’s taking the rest of us so long… 😁