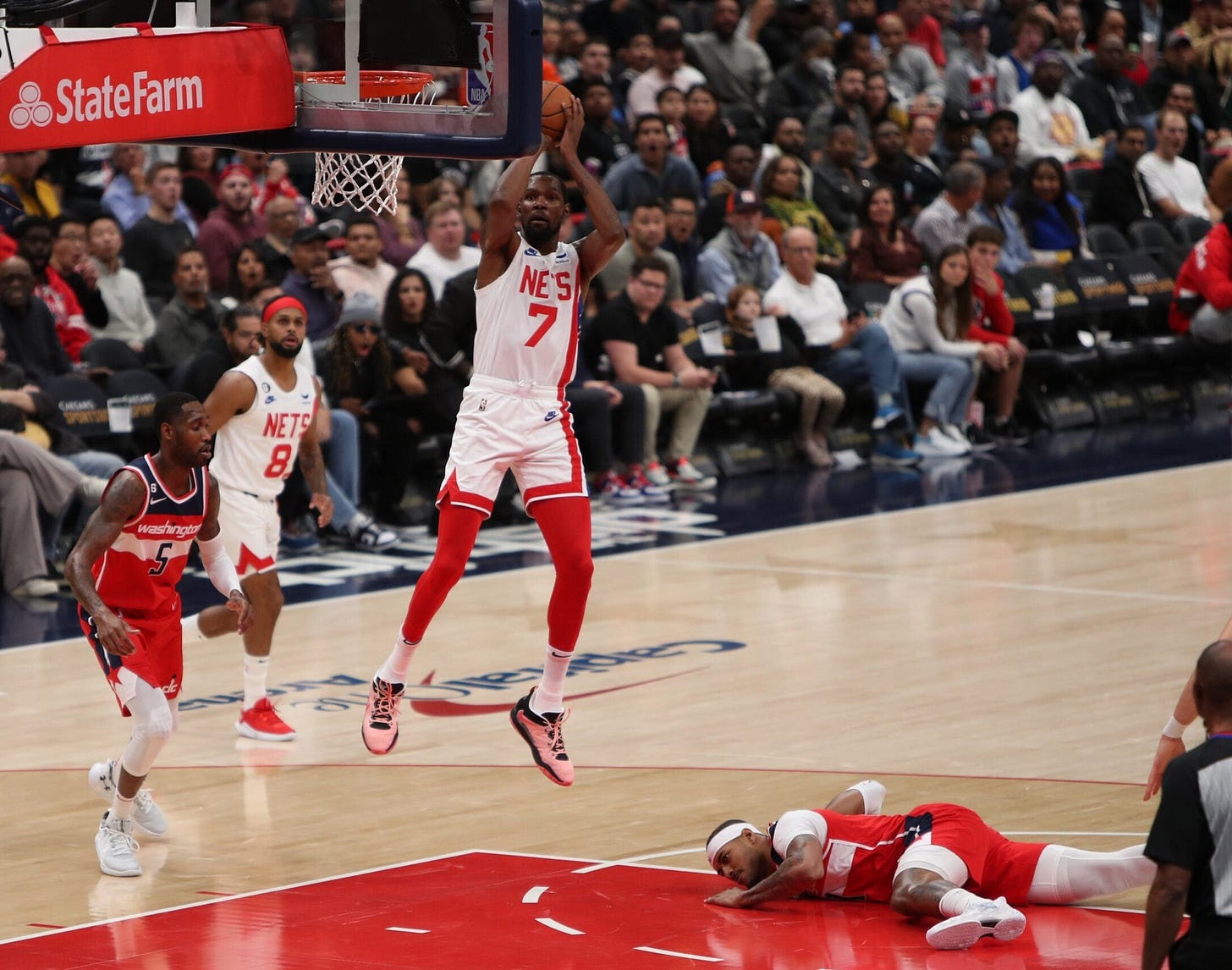
Here's a thing I hadn't thought about before:
If you are the vice president of customer experience at a large airline, you are probably worried about your job. You were hired to affordably provide great customer service to millions of people, and for years, you built a sprawling array of infrastructure to do it. You hired thousands of passenger assistance representatives and reservations agents; you created an assortment of ticketing applications in Microsoft Azure; you recruited a team of engineers to build them in Java and Angular. But, because you believe in continuous innovation, you’ve been following what’s been happening in Silicon Valley. It is the Age of AI; of intelligence; of agents. The building blocks are in place, and it is the dawn of a new era. Though AI will save the world, it might get you, a customer support leader overseeing a worldwide network of call centers, fired.
And so, you are marshalling all the money you can to investigate this new future. “It’s critical for us to be at the forefront of this transformational wave,” you say when you get back from the Airport Customer Experience Summit in Atlanta. “Airlines that adopt generative AI technologies will be able to provide better experiences to their customers, and more value to their shareholders. Airlines that don’t will have worse margins, lower earnings, and more frustrated customers. We cannot be Blockbuster; we must be Netflix.” People nod along, because everyone agreed with you already. You secure a big budget. You go shopping. You buy lots of stuff.
By all accounts, there are thousands of stories like this. In the two years since ChatGPT came out, countless companies have urgently funded innovation budgets. They have created AI skunkworks teams and anointed chief AI officers. They’ve sent emissaries to Silicon Valley to come back with a plan. They have FOBO—Fear of Blockbustering Ourselves—and are ready to spend every dollar they can muster to not be a Harvard Business School case study for the class of 2040.
Though these sorts of things have happened before—there was the internet; there was mobile; there was, in its own little manic way, crypto and web3—this hype cycle seems to be different in two ways.
First—and obviously—it’s all happening at once. The internet and the cloud was a slower burn; the mobile revolution had its doubters; web3 never went mainstream. There were early adopters, late adopters, and Blockbusters. This time around, everything is tighter. Aesthetically, the technological discontinuity is more severe; the pace of development is faster; the coverage is even more breathless; the skeptics are harder to find. There is no adoption curve anymore; there are only users, and the walking dead. And that seems to make the cycle spin faster: Spending creates a sense of momentum, which signals more confidence in AI’s inevitability, which makes everyone more afraid, which makes spending even more necessary.
But here’s the other thing—and this is the dynamic in all of this that seems much less obvious—if you are the vice president of customer experience at a large airline and you now have a big budget to spend on AI, you don’t want to buy it from IBM. The world isn’t being invented by “global innovation centers;” it’s not being invented in office parks where people take 90-minute lunches at Panera; it’s not being invented on PCs, with Excel, on Teams. It’s being invented by the kids.
The spreadsheet people are slow moving. They build product suites, and enterprise SKUs. They package technologies into industry solutions. They give pitches about the necessity of digital transformation, and also talk about shareholder value. Their slides are full of text, in PowerPoint, and have legal disclaimers on the bottom. They aren’t inventing AI, but “investigating it like an anthropologist, or an alien visiting Earth.”1
The kids aren’t investigating. They aren’t bemused. They are hacking, building—without budgets, without spreadsheets, with reckless abandon. The regulators’ whistles don’t stop them; the looming giants don’t deter them; they are accelerating anyway, bulleting down the lane, with no regard for human life. They don’t have slides; they have demos. Their offices are full of vertical monitors covered in code. They are crazy, to the vice president of customer experience at a large airline, but the crazy ones are who will change the world. If you’re afraid of the future, you want to find the people who are building it, not the people who are hosting webinars about their new Modern Intelligence™ offering, now in private preview.
In other words, not only is there no incumbency advantage; if anything, being a large, mature vendor is bad. Normally, Oracle can walk into the office of a major airline, and win on, roughly, vibes: “We understand your enterprise needs; we have the security teams that will make your CIO comfortable; we will hold your hand through this important partnership; our roster of customers includes every other major airline. You are safe with us.” With AI products, the vibes are at least partially inverted. The best vendor is the one that walks in with a futuristic demo, a pile of cash from premier venture capitalists, and the wild eyes of an unhinged visionary.2
Together, these two dynamics seem to be breaking the usual laws of startup growth. Glean, a work automation platform, is making $40 million a year less than four years after its founding. Harvey, an AI lawyer, is making $50 million after only three years. The monthly revenue for Cursor, an AI-coding assistant, went from making $350,000 to $4 million in six months. Eleven Labs, an generative audio company founded in 2022, is making $80 million a year; 11x, a startup building digital workers (and a different company than Eleven Labs), launched in late 2023 and is already approaching $10 million in annual revenue. Those old parabolic curves of the fastest-growing SaaS companies reaching $100 million in revenue in five to seven years now look quaint. Today, the lines are straight up.
When people look at these numbers, they typically say, man, what a great time to start a company.3 And that may be true, in the sense that the world is turning upside down, and there’s lots of money that could eventually be made.
But the “eventually” part seems awfully important. Because in this context—in a market that’s cranking out hundreds of new startups a month, that’s funded by a trillion dollar corporate slush fund, in which the incumbents are cringe, and some companies are regularly going from zero to $60 million in a couple years—what is “traction?” Does a company that’s making $30 million dollars have a real grip on the market?
If you can found a business in 2023, and be making tens of millions of dollars by the end of 2024, someone else can too. Absent some other durable moat—like network effects, superior production process, or unique access to a scarce resource, none of which many AI startups have—most software companies have only two ways to stay ahead of their competition: Brand, and the long slog of putting in more effort. But young brands are fickle, and two years is not a long slog.
Though revenue typically feels like some sort of moat too, that’s mostly because companies historically haven’t been able to make that much money without one. A SaaS startup becomes inevitable once it passes $10 million in annual revenue because you can’t make $10 million without refining a product, warding off legacy competitors, cultivating a sales channel, and trudging through years of messy effort. That was the old scale: $10 million is good progress, $100 million is the ambition, and the less time it takes to get there, the better.
Does that still apply? At what point does the signal from fast growth invert, and no longer implies that a company is taking over some huge market, but instead implies that it found a huge vein of shallow gold in the ground, with no way to defend its mine? If you quietly dig a deep well behind a high wall and find a $100 million deposit of gold, you’re rich. If you stick a shovel in the ground in a crowded field and find a $100 million deposit of gold, you’ve got a problem. The former is a business; the second is a war.
Or, more explicitly, which company has a more promising future:
The AI startup that was founded in 2022, launched in 2023, and all of the sudden makes $20 million?
The AI startup that was founded in 2019, launched 2021, has been refining a product, building a company, and establishing a market presence, and now makes $20 million?
—
When people write warnings about the AI bubble, they tend to fret about the broader ecosystem: The cost of data centers; Nvidia’s stock price; the durability of big LLM providers like OpenAI and Anthropic.4 They wonder if AI will live up to its world-changing promise, or if it will simply be an empty burner that cooks an already overheating world.
It seems like there’s another, more complex bubble building around the peripheral AI companies too. It’s not a bubble that misrepresents the fundamentals—there is real money in automating stuff; these are real companies solving real problems; they aren’t overhyped Ponzi schemes.
But they’re exposed. They’re out in the open. Though 1,000 vice presidents of customer experience throwing their wallets at startups change how quickly companies can grow, they don’t change how long it takes to build a recognizable brand or a rolodex of customer relationships. FOBO compresses the time it takes to make money, but it doesn’t compress the time it takes to build something durable. And a lot of today’s promising companies—and venture dollars—could disappear into that gap.
Pinterest
It’s always been one of the great mysteries of Silicon Valley to me. Facebook and Google became two of the most valuable companies in the world by building giant elaborate services that, in effect, indirectly tricked you into telling them what stuff you wanted to buy so that they could sell that information back to the people who wanted to sell it to you. Google parses thousands of your searchers, cleverly derives that you are a 27-year-old girl living in Dimes Square, and sells that conclusion to New Balance so that they can send you an ad for their new “Snoafer.”
Of course, sometimes Google gets it wrong. Sometimes they think you’re a 27-year-old girl living in Dimes Square who would like the Snoafer, and you are; sometimes they think that, and you’re a man in Indiana who just watches a lot of Outer Banks, and you do not like the Snoafer.
Pinterest skips the middleman. There is no algorithm; there are no searches about “how to host a friendsgiving in a small apartment” that get probabilistically transformed into a likely Snoafer buyer. On Pinterest, you just tell it you want the Snoafer. That’s the whole thing: You look at stuff, and you pin the stuff you want to buy. It’s the quiet part of every consumer internet company said out loud. And 500 million people use it every month.
Given that Facebook and Google’s roundabout version of the same product has made them two of the most valuable companies on the planet, how is Pinterest not also huge? How is it not its own empire? How come we don’t talk about the FANGAP instead of the FAANGs?
But maybe I’ve got it backwards. From Ryan Broderick’s Garbage Day:
Almost every major Chinese social app is built around “social shopping”. This is why they all emphasize trends over viral one-offs. They want you to buy a product and make content with it to inspire everyone else to make content with it. This is also why they hyper-target your interests so aggressively. But because Americans have no experience with these kind of apps, the impact of TikTok’s algorithm has been different here. Sure, there’s plenty of shopping — Stanley Cups are probably the best, most recent example of the TikTok e-commerce effect. But, as WIRED recently point out, those systems have, perhaps inadvertently, been mainly used in the US to create genuinely supportive filter bubbles for young people, for different subcultures, strange fandoms, and all kinds of other communities. Something western companies like Meta have not ever been able to crack, possibly because, ironically enough, they aren’t nearly as focused on directly selling you shit, and much more interested in selling you to advertisers. And this irony is even more pronounced now that TikTokers are migrating to RedNote, which is, yes, like Pinterest or Instagram, but could more accurately be compared to QVC.
In the United States, we build social networks to collect data that we can sell to advertisers. According to Broderick, in China, people build apps to sell you stuff, then figure out who you are and the types of videos you might like based on that. And because the stuff you want to buy so precisely defines the types of communities you exist in,5 content curated by your shopping list is actually more engaging than content curated by some social graph.
So maybe Pinterest is actually even more valuable than I thought—not as a smarter source of advertising data, but as the next new social network. Which works out, because we might need one soon.
Drudgery
People at tech companies often refer to their computers as their “machine.” There’s something mildly appropriative to it, as if we are operating a jackhammer or a plow. But the industrial connotation is, I think, intentional: It reminds us that we are humans, who are creative and thoughtful; the machine is our creativity, levered. We are the management, and it is the labor.
I’ve wondered before if AI undoes this relationship:
This pattern—computers automating more and more mechanical work; people doing more and more creative work—has become our default assumption about how technology advances, and for what we should do with new technology. …
But this doesn’t have to continue forever. Technology could—again, in a very rough sense—go the other way. It could be more creative than reliable. It could subsume our lives from the top down, outperforming us in strategic tasks while struggling with the tactical ones. If this happens, the best things we could build with it might impose a choice: Do we use it to make us better at something, or do we use it to replace something we don’t want to do?
Tyler Cohen sees the same inversion coming for academia:
AI will know almost all of the academic literature, and will be better at modeling and solving most of the quantitative problems. It will be better at specifying the model and running through the actual statistical exercises. Humans likely will oversee these functions, but most of that will consist of nodding, or trying out some different prompts.
The humans will gather the data.
Most predictions about AI are hyperbolic: It will destroy humanity, or it will usher an era of unprecedented prosperity. In twenty years, we will all be dead, or we will all be living lives of uninterrupted leisure. It will hunt us for sport and algorithmic pleasure, or it will do the repetitive and boring things we don’t like and “free up human capacity for more meaningful work.” We will either be carefree philosophers and creatives, or an electrolyte goo.
The truth may somehow be worse: Rather than turning us into batteries, the machines will make us Jira admins.
SDF
Now, this low-friction workload portability doesn’t happen automatically just because you have an open file format, table format, and metastore. From what I can tell, in order to make this a reality, you need:
An ability to transpile workloads between execution engines’ dialects / environments with accuracy guarantees.
An ability to route workloads automatically between multiple execution engines.
An ability to decision which engine is best suited to execute a given workload.
The platforms themselves have to have a minimum shared level of support for the various table formats and metastores, with appropriate performance characteristics.
The ideal database would not only separate buckets [i.e., storage] and calculators [i.e., compute engines], but it would also separate the calculators from the programs that people want to run on them.
Because, today, in order to use multiple calculators, you have to write programs in multiple languages. Every database engine has different APIs and uses a different variants of SQL, and all the queries and pipelines and applications built on that engine need to use that variant. You can’t simply point a query that was originally written for Databricks at a Snowflake or a DuckDB engine, because there are very stupid differences between all three of them. Even if the calculators are interoperable with the buckets, the programs are not interoperable with the calculators.
So that seems like what’s next—dbt directly on top of S3, more or less. You write queries in one language, like dbt SQL or SDF SQL, and it gets rewritten in whatever version of SQL a specific execution engine prefers. The programs and pipelines people write would then be agnostic to the calculators underneath them—which would then, as Tristan proposes, make it possible for people to choose the right engine for the right job.
dbt Labs has acquired SDF Labs. …
SDF is a high performance toolchain for SQL development packaged into one CLI; a multi-dialect SQL compiler, type system, transformation framework, linter, and language server.
I can’t link to it, but this line comes from the lede in a recent New Yorker email for this article about someone trying TikTok for the first time. Look, I appreciate the New Yorker, and would definitely work for the New Yorker (that font, it just looks expensive*), but, what? It’s 2025! TikTok is eight years old! It’s hours from getting banned! The kids are moving on! And the New Yorker sending a child into the middle of the movie to find out what’s going on? And the thing they find is that they’re “amused by the algorithm’s tailor-made content?” What are we doing here???
* The cut from the Bieber quote straight to the Skrillex quote, just savage.
Or, even better, you walk in with the swagger of a Fortune 500 CEO, the technological credentials of a CTO, the network of a professional board member, the money of a billionaire, and the entrepreneurial track record of someone who should be retired in the French Riviera. That person can probably close some deals too.
Of course, in this case, the person saying it is the person who collects 20 percent of every one of these startups’ revenues.
TechCrunch recently reported that Facebook AI team’s wanted to better model than GPT-4:
“Our goal needs to be GPT-4,” said Meta’s VP of Generative AI, Ahmad Al-Dahle, in an October 2023 message to Meta researcher Hugo Touvron. “We have 64k GPUs coming! We need to learn how to build frontier and win this race.”
On one hand, whatever; this is just internal chatter about wanting to build something good; it is not, as TechCrunch put it, execs being obsessed with beating ChatGPT. On the other hand, this sort of clock speed comparison, in which major LLM providers are willing to spend more and more money to nudge ahead, is exactly what makes them seem economically untenable. How long can you keep incinerating cash to make improvements that will probably be available for a fraction of the cost six to twelve months later? How much is being at the front of the peloton about being cool rather than rational?
I don’t want to think too hard about what that implies.
"The truth may somehow be worse: Rather than turning us into batteries, the machines will make us Jira admins."
I have never considered Pinterest vs other tech company algorithms/business models. One would think Amazon would know a thing or two about the "sell people stuff" model and possibly create a platform for social selling. Maybe they missed their chance with Vine back in the day. 😏